AIxiv專欄是本站發布學術、技術內容的欄位。過去數年,本站AIxiv專欄接收通報了2,000多篇內容,涵蓋全球各大專院校與企業的頂尖實驗室,有效促進了學術交流與傳播。如果您有優秀的工作想要分享,歡迎投稿或聯絡報道。投稿信箱:liyazhou@jiqizhixin.com;zhaoyunfeng@jiqizhixin.com
上海交通大學生成式模型實驗室(GAIR Lab) 的研究團隊,主要研究方向與大模型實驗室(GAIR Lab) 的研究團隊,主要研究方向為大模型評估。 AI技術日新月異,近來Anthropic公司最新發布的Claude-3.5-Sonnet因在知識型等任務上設立新產業基準而引發廣泛討論:Claude-3.5-Sonnet 已經取代OpenAI的GPT4o成為世界上」最聰明的AI「(Most Intelligent AI)了嗎? 回答這個問題的挑戰在於我們首先需要一個足夠挑戰的智力測驗基準,使得我們可以區分目前最高水準的AI。 上海交通大學生成式人工智慧實驗室(GAIR Lab)推出的OlympicArena[1] (奧林匹克競技場)滿足了這個需求。 奧林匹克學科競賽不僅是對人類(碳基智能)思維敏捷性、知識掌握和邏輯推理的極限挑戰,更是AI(「矽基智能」)鍛鍊的絕佳練兵場,是佳練兵場,是衡量AI與「超級智慧」距離的重要標尺。 OlympicArena-一個真正意義上的AI奧運競技場。在這裡,AI不僅要展現其在傳統學科知識上的深度(數學、物理、生物、化學、地理等頂級競賽),還要在模型間的認知推理能力上展開較量。
近日,同樣是研究團隊,首次提出使用"奧林匹克競賽獎牌榜"的方法,根據各AI模型在奧林匹克競技場(各學科)的綜合表現進行排名,選出迄今為止智力最高的AI。在此次競技場中,研究團隊重點分析並比較了最近發布的兩個先進模型-Claude-3.5-Sonnet和Gemini-1.5-Pro,以及OpenAI的GPT-4系列(e.g., GPT4o )。透過這種方式,研究團隊希望能夠更有效地評估和推動AI技術的發展。 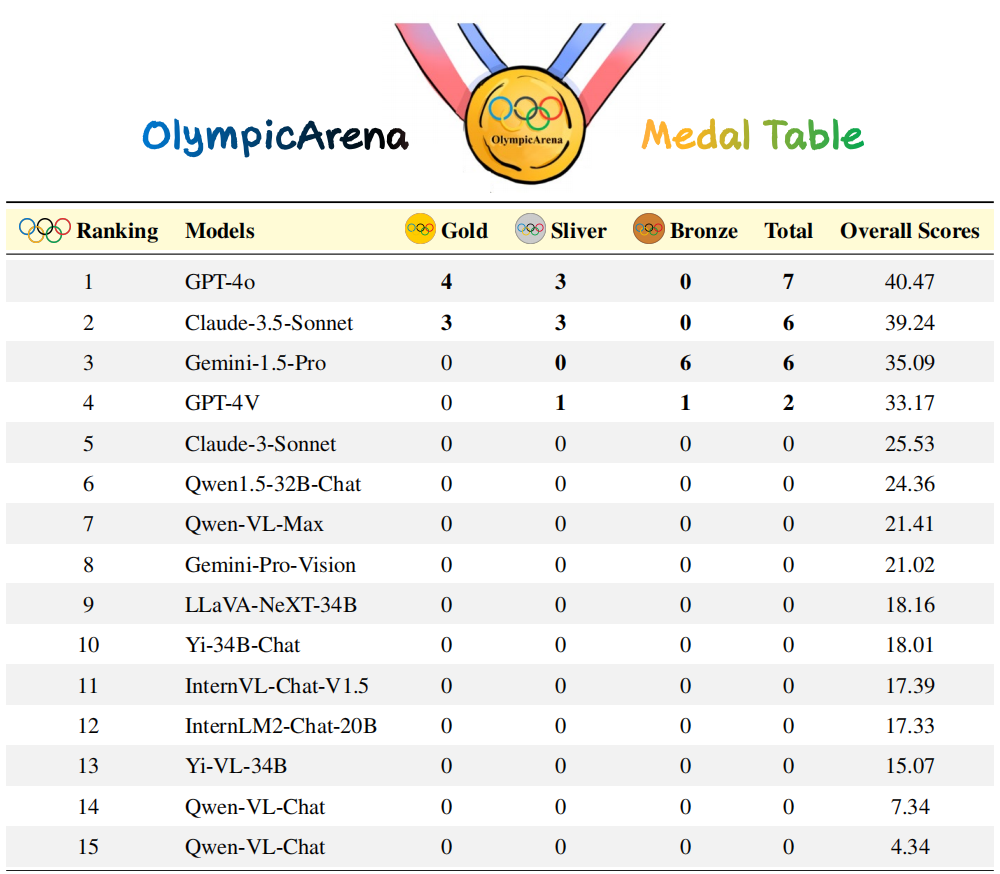
注意:研究團隊首先依據金牌數量對模型進行排序,如果金牌數量相同,則依照整體表現分數來排序。 Claude-3.5-Sonnet在整體表現上與GPTo-4oo
Claude-3.5-Sonnet在整體表現上與GPTo一樣(例如在物理、化學和生物學上)。
- Gemini-1.5-Pro和GPT-4V排名緊隨GPT-4o和Claude-3.5-Sonnet之後,但它們之間存在明顯的表現差距。
- 來自開源社群的AI模型表現明顯落後於這些專有模型。
- 這些模型在此基準測試上的表現不盡人意,顯示我們在實現超級智慧之路上還有很長的路要走。
- Project homepage: https://gair-nlp.github.io/OlympicArena/
The research team took the test set of OlympicArena for evaluation. The answers to this test set are not made public to help prevent data leakage and thus reflect the true performance of the model. The research team tested multimodal large models (LMMs) and text-only large models (LLMs). For testing of LLMs, no image-related information is provided to the model as input, only text. All assessments use zero-shot Chain of Thought prompt words. The research team evaluated a series of open and closed source multimodal large models (LMMs) and text-only large models (LLMs). For LMMs, closed-source models such as GPT-4o, GPT-4V, Claude-3-Sonnet, Gemini Pro Vision, Qwen-VL-Max, etc. were selected. In addition, LLaVA-NeXT-34B, InternVL-Chat-V1.5 were also evaluated. , Yi-VL-34B and Qwen-VL-Chat and other open source models. For LLMs, open source models such as Qwen-7B-Chat, Qwen1.5-32B-Chat, Yi-34B-Chat, and InternLM2-Chat-20B were mainly evaluated. In addition, the research team specifically included the newly released Claude-3.5-Sonnet as well as Gemini-1.5-Pro and compared them with the powerful GPT-4o and GPT-4V. to reflect the latest model performance. Metrics Given that all problems can be evaluated by rule-based matching, the research team used accuracy for non-programming tasks and unbiased pass@k metrics for programming tasks , defined as follows: 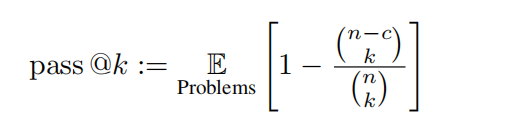
In this evaluation, k = 1 and n = 5 are set, and c represents the number of correct samples that pass all test cases. Olympic Arena Medal List: Similar to the medal system used in the Olympic Games, it is a pioneering ranking mechanism specifically designed to evaluate the performance of AI models in various academic fields. The table awards medals to models that achieve the top three results in any given discipline, providing a clear and competitive framework for comparing different models. The research team first sorted the models according to the number of gold medals. If the number of gold medals was the same, they were sorted according to the overall performance score. It provides an intuitive and concise way to identify leading models in different academic fields, making it easier for researchers and developers to understand the strengths and weaknesses of different models. The research team also conducts accuracy-based fine-grained assessment based on different disciplines, different modalities, different languages, and different types of logical and visual reasoning abilities. The analysis content mainly focuses on Claude-3.5-Sonnet and GPT-4o, and also partially discusses the performance of Gemini-1.5-Pro. 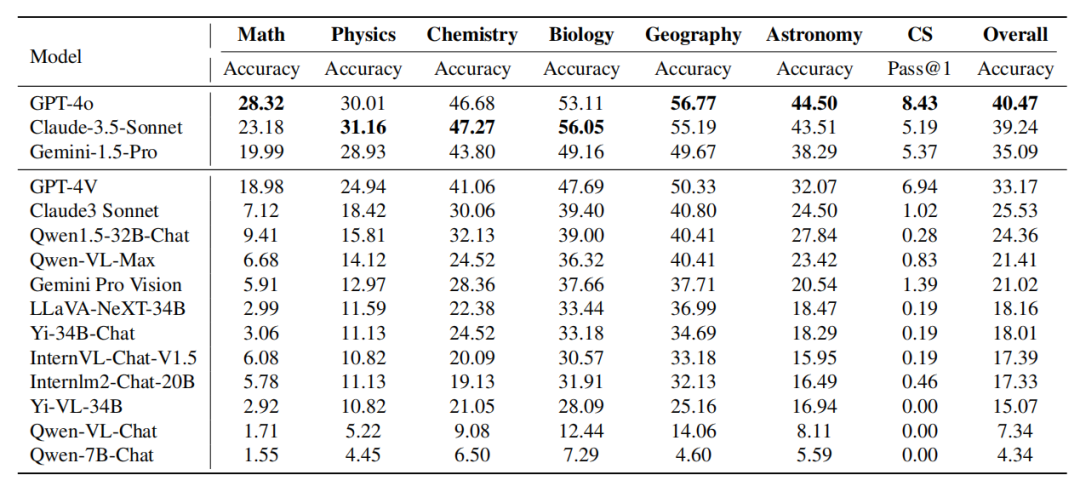
Table: Performance of the model on different subjects The performance of Claude-3.5-Sonnet is powerful and reaches almost Comparable to GPT-4o. The overall accuracy difference between the two is only about 1%. The newly released Gemini-1.5-Pro has also shown considerable strength, outperforming GPT-4V (OpenAI’s current second most powerful model) in most disciplines.
It’s worth noting that at the time of writing, the earliest of these three models was released only a month ago, reflecting the rapid development in this field. - Fine-grained analysis for disciplines
GPT-4o vs. Claude-3.5-Sonnet:
Although GPT-4o and Claude-3. 5-Sonnet in the whole The performance is similar, but both models exhibit different subject advantages. GPT-4o demonstrates superior capabilities on traditional deductive and inductive reasoning tasks, especially in mathematics and computer science. Claude-3.5-Sonnet performs well in subjects such as physics, chemistry, and biology, especially in biology, where it exceeds GPT-4o by 3%.
GPT-4V vs. Gemini-1.5-Pro: A similar phenomenon can be observed in the comparison of Gemini-1.5-Pro vs. GPT-4V. Gemini-1.5-Pro significantly outperforms GPT-4V in physics, chemistry, and biology. However, in terms of mathematics and computer science, Gemini-1.5-Pro's advantages are not obvious or even inferior to GPT-4V. From these two sets of comparisons, it can be seen that: OpenAI’s GPT series performs outstandingly in traditional mathematical reasoning and programming capabilities. This shows that the GPT series models have been rigorously trained to handle tasks that require a lot of deductive reasoning and algorithmic thinking. In contrast, other models such as Claude-3.5-Sonnet and Gemini-1.5-Pro demonstrated competitive performance when it comes to subjects that require combining knowledge with reasoning, such as physics, chemistry, and biology. This reflects the areas of expertise and potential training focus of different models, indicating possible trade-offs between reasoning-intensive tasks and knowledge integration tasks. Fine-grained analysis of reasoning types
- Caption: The performance of each model in terms of logical reasoning capabilities. Logical reasoning abilities include: deductive reasoning (DED), inductive reasoning (IND), abductive reasoning (ABD), analogical reasoning (ANA), causal reasoning (CAE), critical thinking (CT), decomposition reasoning (DEC) and quantitative Reasoning (QUA).
Comparison between GPT-4o and Claude-3.5-Sonnet in terms of logical reasoning capabilities: 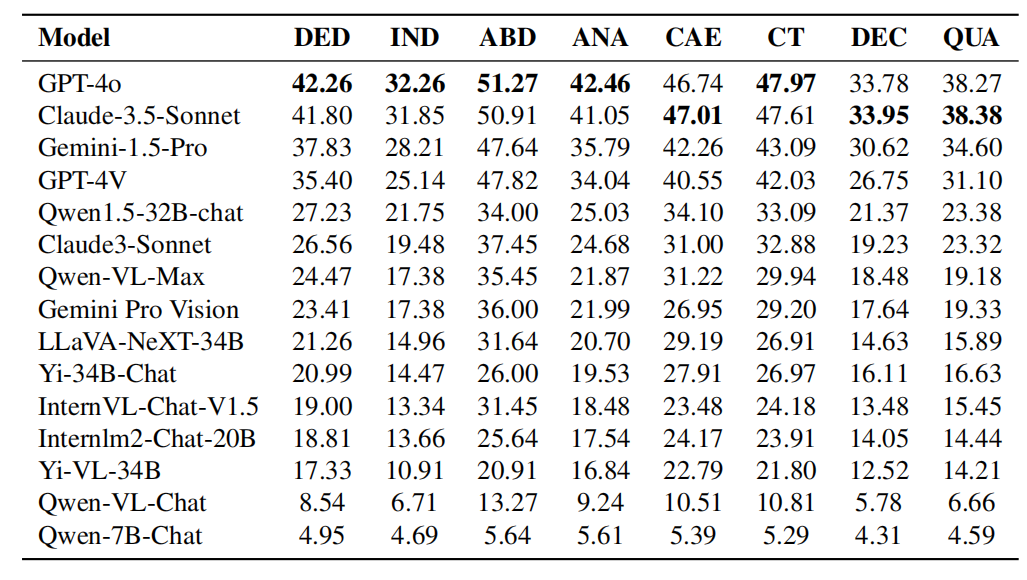
As can be seen from the experimental results in the table, GPT-4o has excellent performance in most logical reasoning capabilities Better than Claude-3.5-Sonnet in areas such as deductive reasoning, inductive reasoning, abductive reasoning, analogical reasoning and critical thinking. However, Claude-3.5-Sonnet outperforms GPT-4o in causal reasoning, decomposition reasoning, and quantitative reasoning. Overall, the performance of both models is comparable, although GPT-4o has a slight advantage in most categories. Table: Performance of each model in visual reasoning capabilities. Visual reasoning abilities include: pattern recognition (PR), spatial reasoning (SPA), diagrammatic reasoning (DIA), symbolic interpretation (SYB), and visual comparison (COM). GPT-4o vs. Claude-3.5-Sonnet Performance in visual reasoning ability: As can be seen from the experimental results in the table, Claude-3.5-Sonnet is better in pattern recognition and Leading in diagram reasoning, demonstrating its competitiveness in pattern recognition and interpretation of diagrams. The two models performed comparably on symbol interpretation, indicating that they have comparable abilities in understanding and processing symbolic information. However, GPT-4o outperforms Claude-3.5-Sonnet in spatial reasoning and visual comparison, demonstrating its superiority on tasks that require understanding spatial relationships and comparing visual data. Comprehensive analysis of disciplines and reasoning types, the research team found that: - Mathematics and computer programming emphasize complex deductive reasoning skills and rule-based derivation of universal conclusions, and tend to rely less on pre-existing Knowledge. In contrast, disciplines like chemistry and biology often require large knowledge bases to reason based on known information about causal relationships and phenomena. This suggests that while mathematical and programming abilities are still valid indicators of a model's reasoning ability, other disciplines better test a model's ability to reason and problem analyze based on its internal knowledge.
- The characteristics of different disciplines indicate the importance of customized training data sets. For example, to improve model performance in knowledge-intensive subjects such as chemistry and biology, the model needs extensive exposure to domain-specific data during training. In contrast, for subjects that require strong logic and deductive reasoning, such as mathematics and computer science, models can benefit from training focused on purely logical reasoning.
- Furthermore, the distinction between reasoning ability and knowledge application demonstrates the potential of the model for cross-disciplinary application. For example, models with strong deductive reasoning capabilities can assist fields that require systematic thinking to solve problems, such as scientific research. And knowledge-rich models are valuable in disciplines that rely heavily on existing information, such as medicine and environmental science. Understanding these nuances helps develop more specialized and versatile models.
Fine-grained analysis of language types
Caption: The performance of each model in different language problems.
The above table shows the performance of the model in different languages. The research team found that most models were more accurate on English than on Chinese, with this gap being particularly significant among the top-ranked models. It is speculated that there may be several reasons: - Although these models contain a large amount of Chinese training data and have cross-language generalization capabilities, their training data is mainly English-based.
- Chinese questions are more challenging than English questions, especially in subjects like physics and chemistry, Chinese Olympiad questions are harder.
- These models are insufficient in identifying characters in multi-modal images, and this problem is even more serious in the Chinese environment.
However, the research team also found that some models developed by Chinese manufacturers or fine-tuned based on base models that support Chinese perform better in Chinese scenarios than in English scenarios, such as Qwen1.5-32B-Chat, Qwen -VL-Max, Yi-34B-Chat and Qwen-7B-Chat, etc. Other models, such as InternLM2-Chat-20B and Yi-VL-34B, while still performing better in English, have much smaller accuracy differences between English and Chinese scenes than the top-ranked closed-source models. many. This shows that optimizing models for Chinese data and even more languages around the world still requires significant attention. Fine-grained analysis of modalities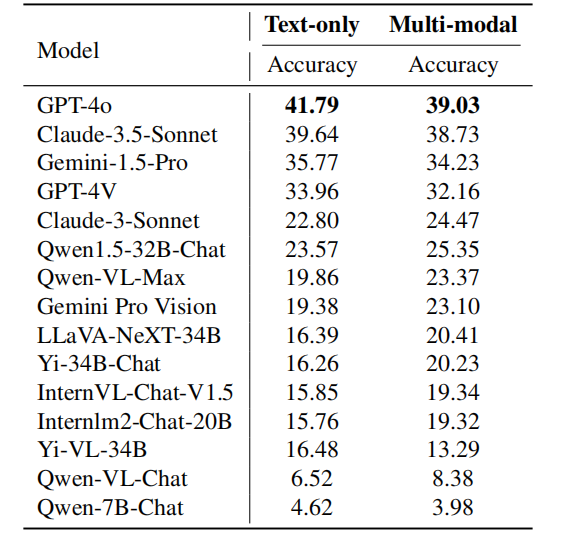
to to to to to to to to to to solve different modal problems to be solved. The above table shows the performance of the model in different modalities. GPT-4o outperforms Claude-3.5-Sonnet in both plain text and multi-modal tasks, and performs more prominently on plain text. On the other hand, Gemini-1.5-Pro performs better than GPT-4V on both plain text and multi-modal tasks. These observations indicate that even the strongest models currently available have higher accuracy on text-only tasks than on multi-modal tasks. This shows that the model still has considerable room for improvement in utilizing multi-modal information to solve complex reasoning problems. In this review, the research team mainly focused on the latest models: Claude-3.5-Sonnet and Gemini-1.5-Pro, and compared them with OpenAI's GPT-4o and GPT- 4V for comparison. In addition, the research team also designed a novel ranking system for large models, the OlympicArena Medal Table, to clearly compare the capabilities of different models. The research team found that GPT-4o excels in subjects such as mathematics and computer science, and has strong complex deductive reasoning capabilities and the ability to draw general conclusions based on rules. Claude-3.5-Sonnet, on the other hand, is better at reasoning from established causal relationships and phenomena. In addition, the research team also observed that these models performed better on English language problems and had significant room for improvement in multi-modal capabilities. Understanding these nuances of models can help develop more specialized models that better serve the diverse needs of different academic and professional fields. As the quadrennial Olympic event approaches, we can’t help but imagine what kind of peak showdown between wisdom and technology it will be if artificial intelligence can also participate? It is no longer just a physical competition. The addition of AI will undoubtedly open up a new exploration of the limits of intelligence. We also look forward to more AI players joining this intellectual Olympics. [1] Huang et al., OlympicArena: Benchmarking Multi-discipline Cognitive Reasoning for Superintelligent AI https://arxiv.org/abs/2406.12753v1
The above is the detailed content of Choosing the smartest AI in the Olympiad: Claude-3.5-Sonnet vs. GPT-4o?. For more information, please follow other related articles on the PHP Chinese website!