With the rapid development of the Internet, people are faced with a large amount of information and product choices, and personalized recommendations have become an effective means to solve the problem of information overload. As a hot topic in the field of artificial intelligence, deep learning technology has shown strong potential in recommendation systems, providing users with more accurate and personalized recommendation services, and promoting the new frontier of recommendation systems
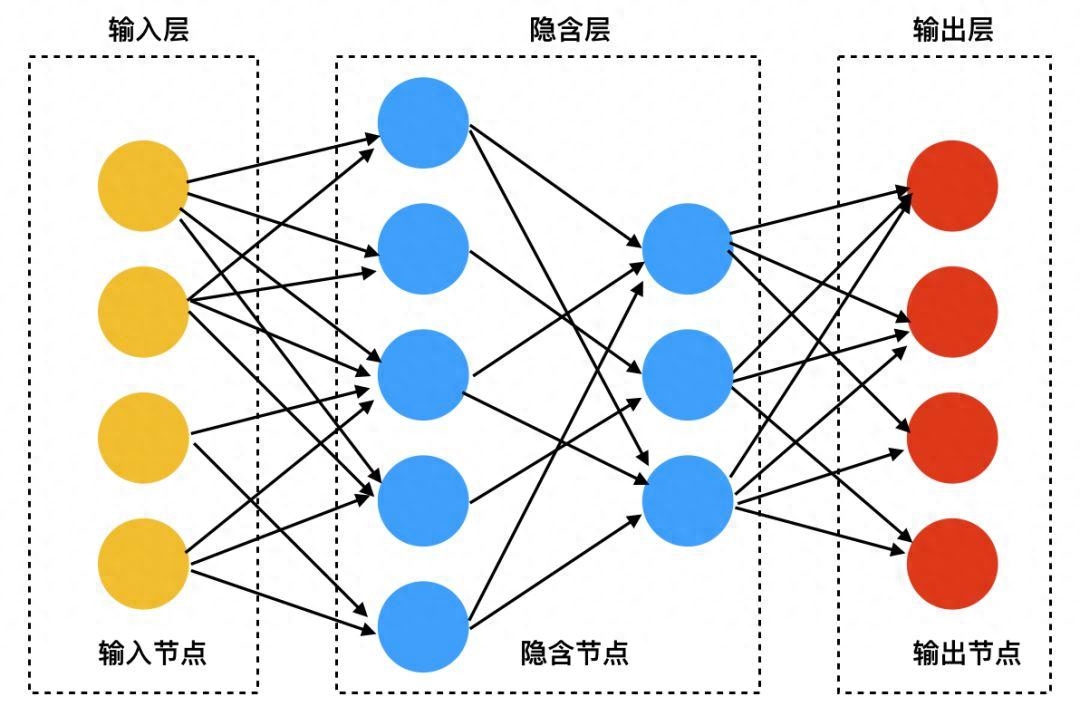
The advantages of deep learning in recommendation systems
- Rich feature representation: Deep learning can automatically learn high-level features of data Abstract features to more accurately capture the relationship between users and items. Traditional recommendation algorithms may require hand-designed features, while deep learning can learn richer and more complex feature representations from data.
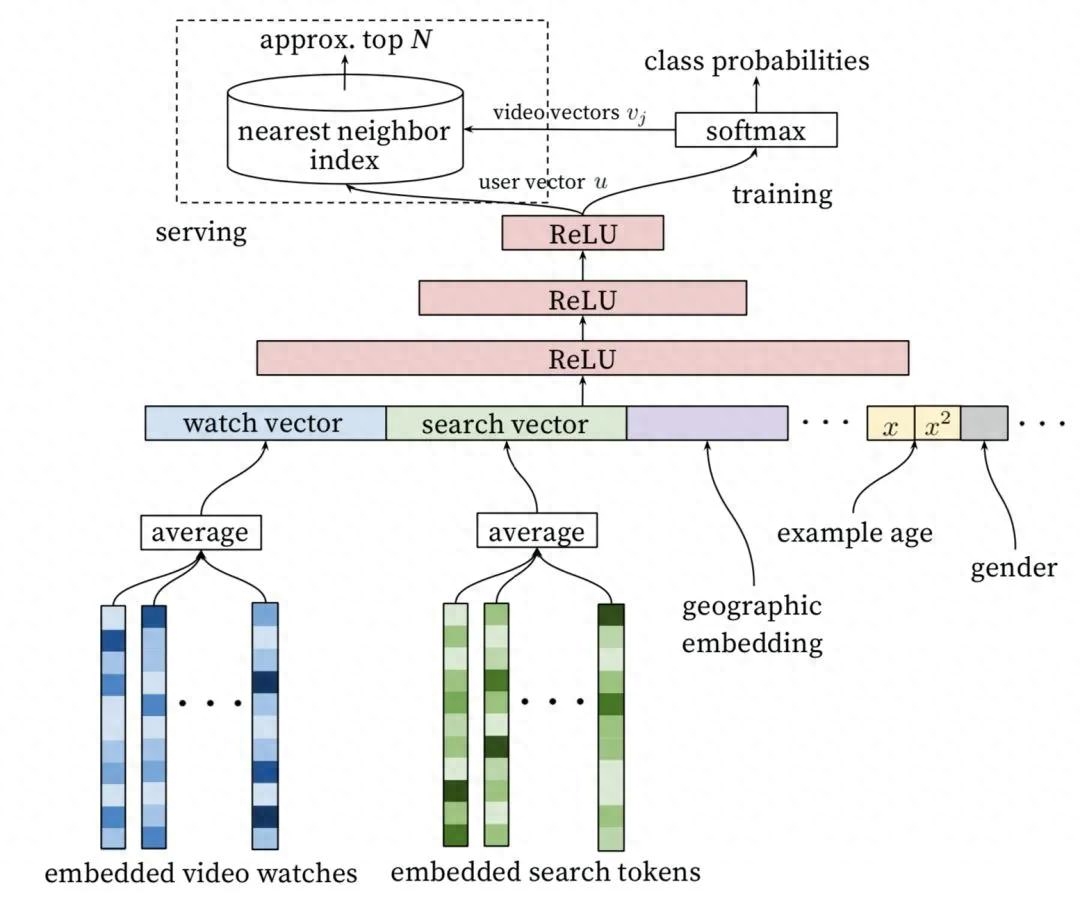
- Implicit correlations: Deep learning can mine implicit correlations in data, not only considering explicit correlations User behavior can also analyze implicit interests and concerns. This makes the recommendation system better able to meet the personalized needs of users.
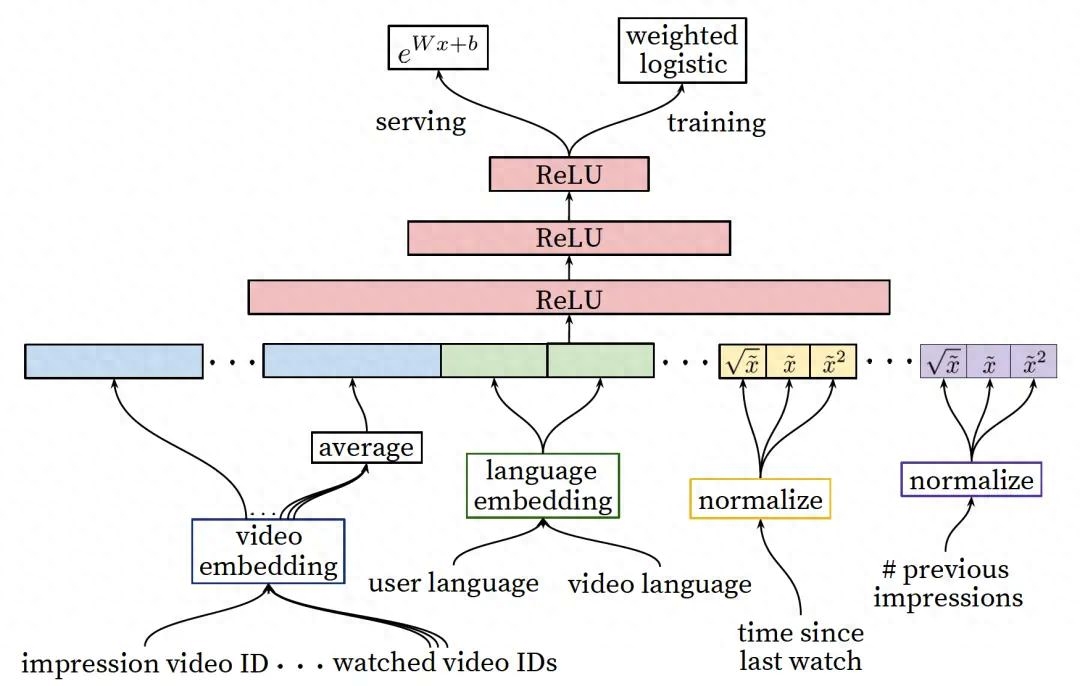
- Scalability of the model: Deep learning models are highly scalable and can adapt to different scales and complexities Recommended scenarios. This gives deep learning great advantages in large-scale recommendation systems.
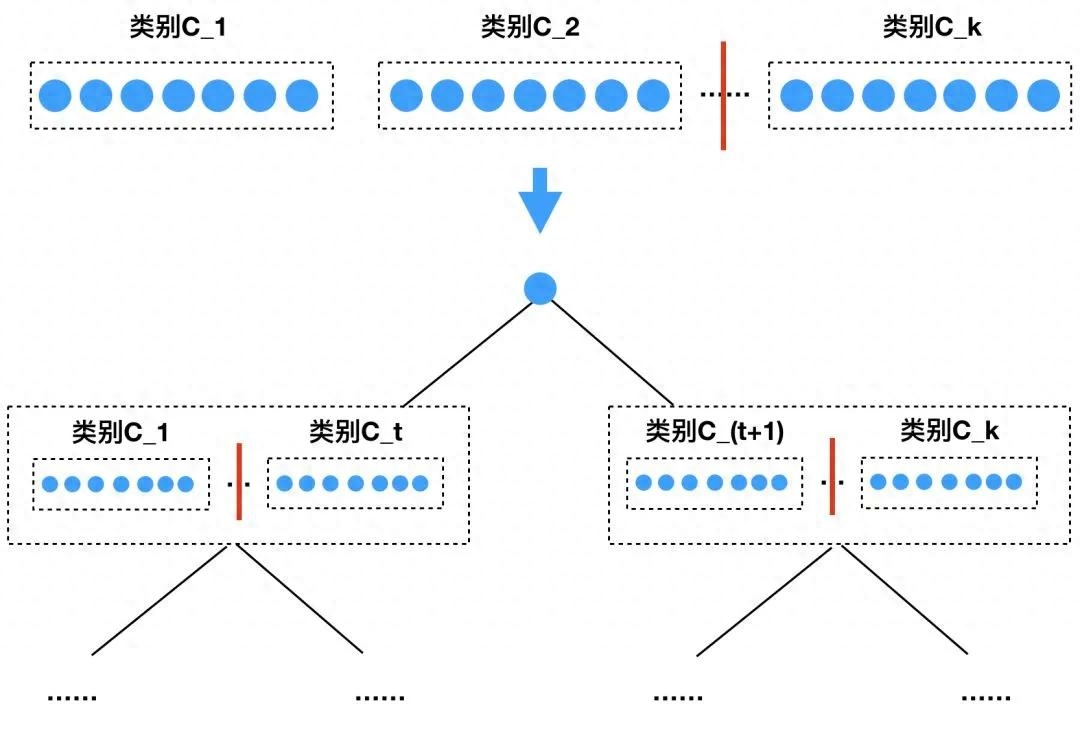
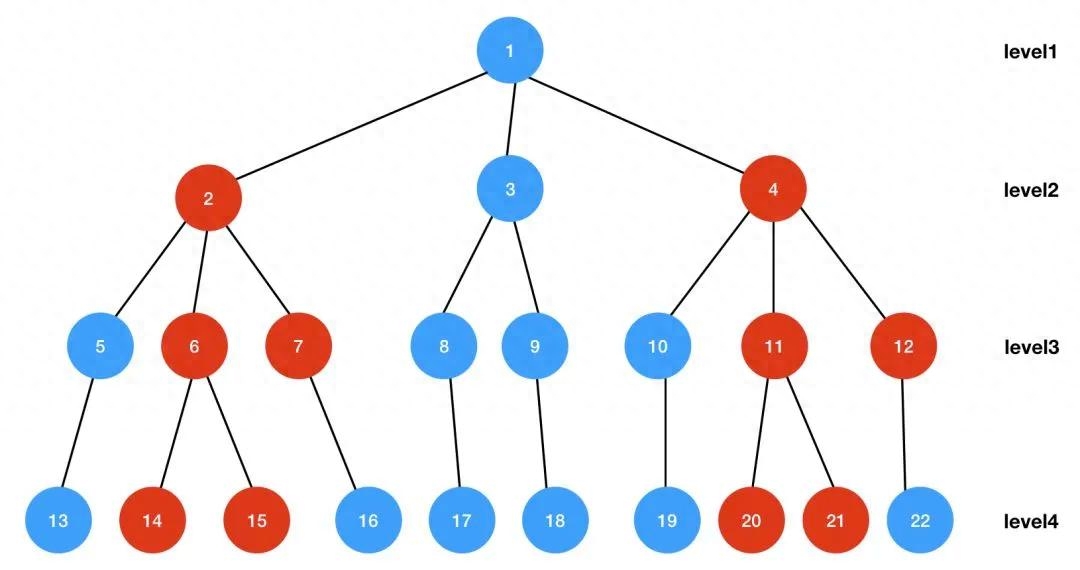
##Application of Deep Learning in Recommendation Systems
- Convolutional Neural Network (CNN): In scenes such as images and texts, CNN is used to learn more effective feature representations. In the recommendation system, CNN can be used to process pictures or text information of products to improve the representation ability of items.
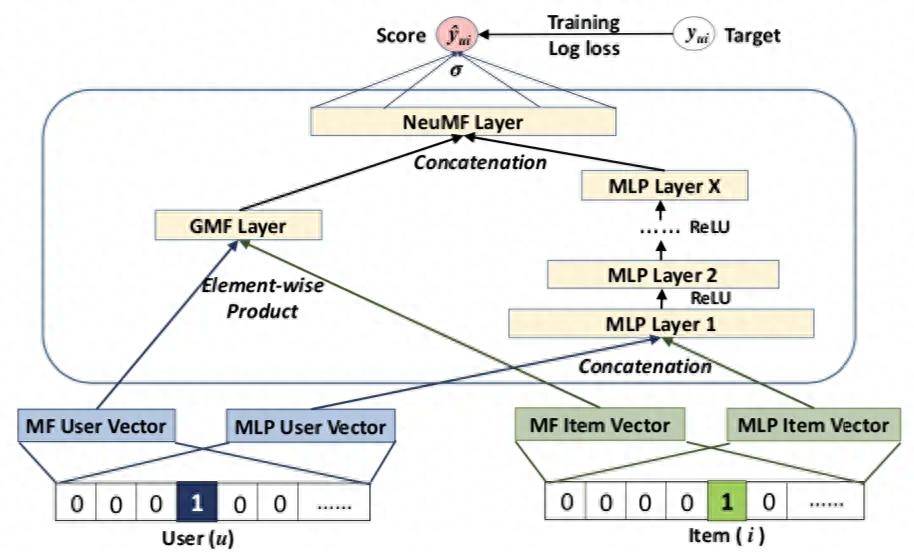
- Recurrent Neural Network (RNN): RNN performs well in sequence data analysis, for The analysis of user behavior sequences has unique advantages. In the recommendation system, RNN can be used to model the user's historical behavior sequence to make more accurate personalized recommendations.
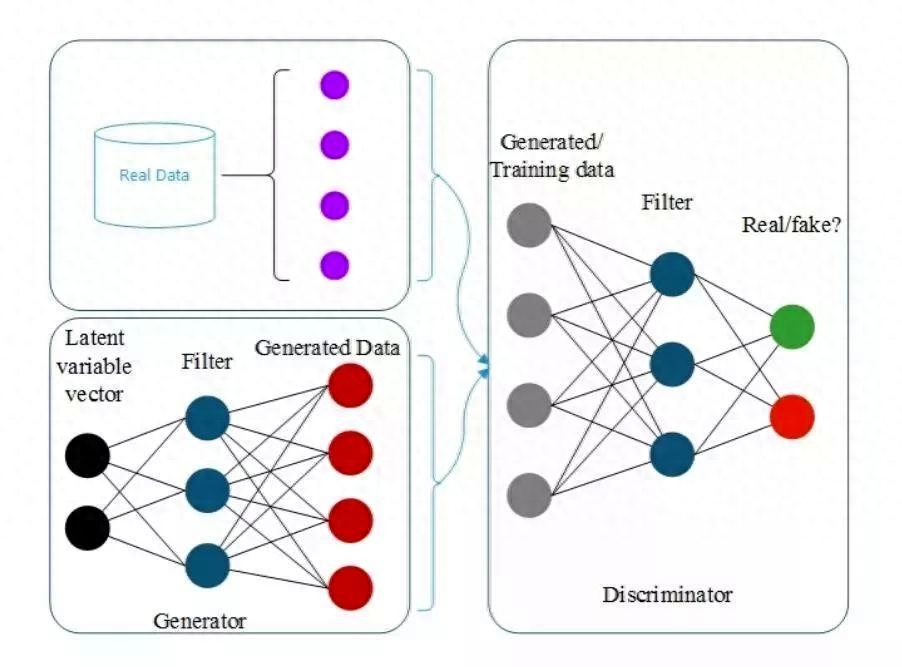
- Deep Matrix Factorization: Combining matrix factorization with deep learning allows you to build more complex models that capture users and items multi-level relationships between them. This has wide applications in recommender systems.
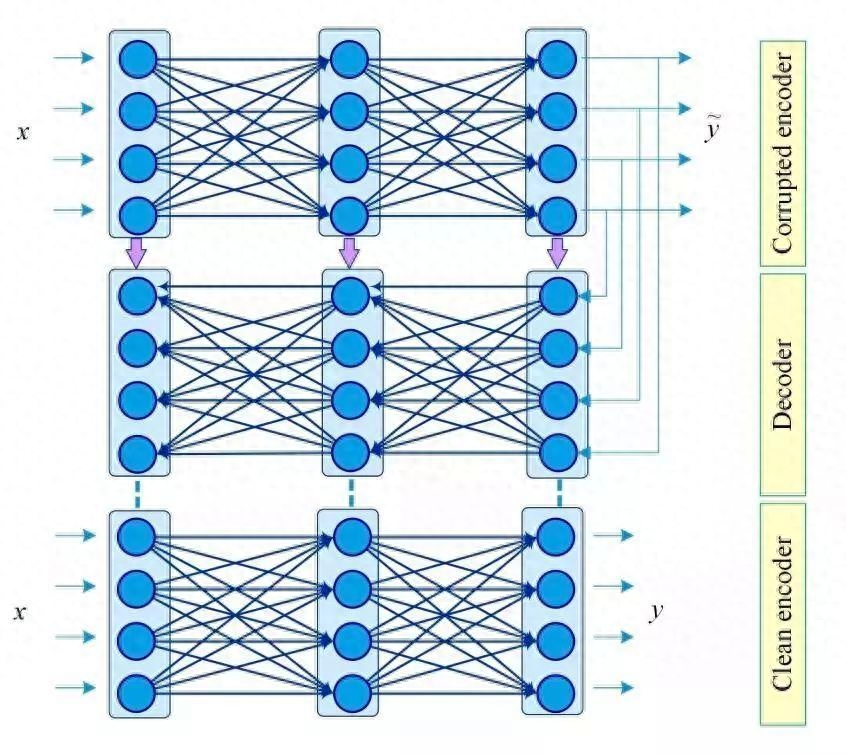
Future Development Trend
With the continuous advancement and promotion of deep learning technology , the application of deep learning in recommendation systems will become more extensive and in-depth. In the future, we can look forward to more innovations and breakthroughs, and more efficient and accurate personalized recommendations will become possible
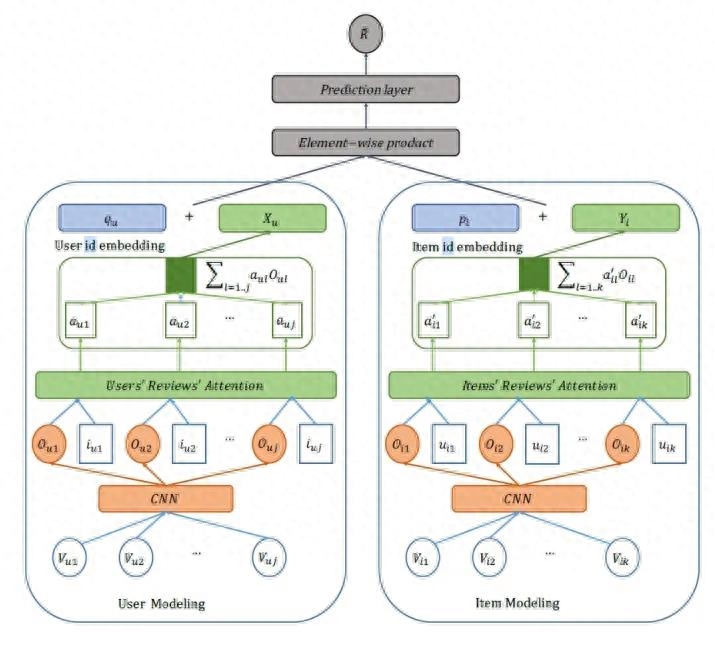
At the same time, as users The requirements for privacy protection and model interpretability continue to increase, and research on deep learning models in these aspects will become increasingly important. Developing a more privacy-preserving and interpretable deep learning recommendation model will become one of the future research directions.
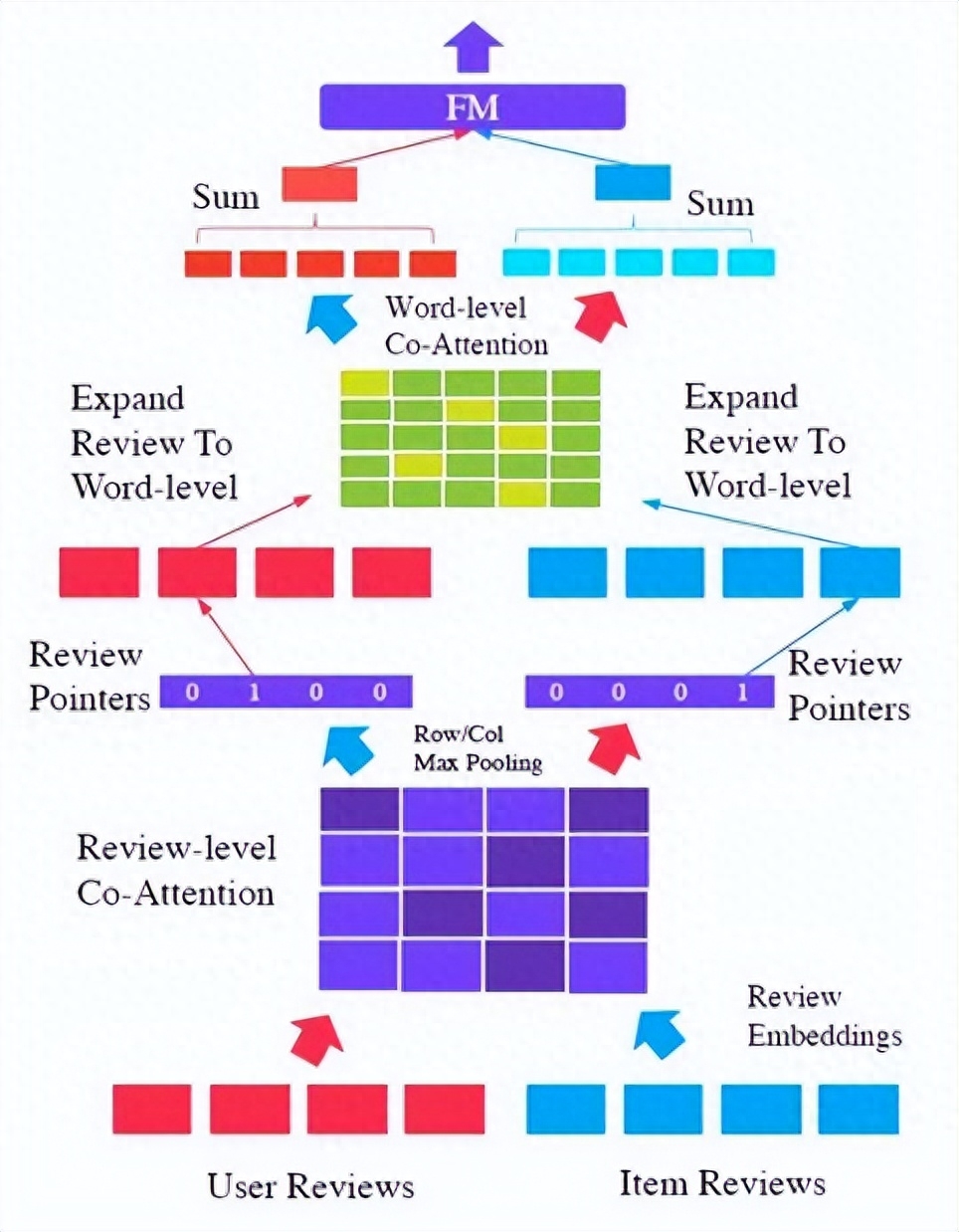
#The application of deep learning in recommendation systems has shown great potential. Through deep learning, we can build a smarter and more personalized recommendation system, provide users with more valuable recommendation services, and also promote new developments in recommendation system research
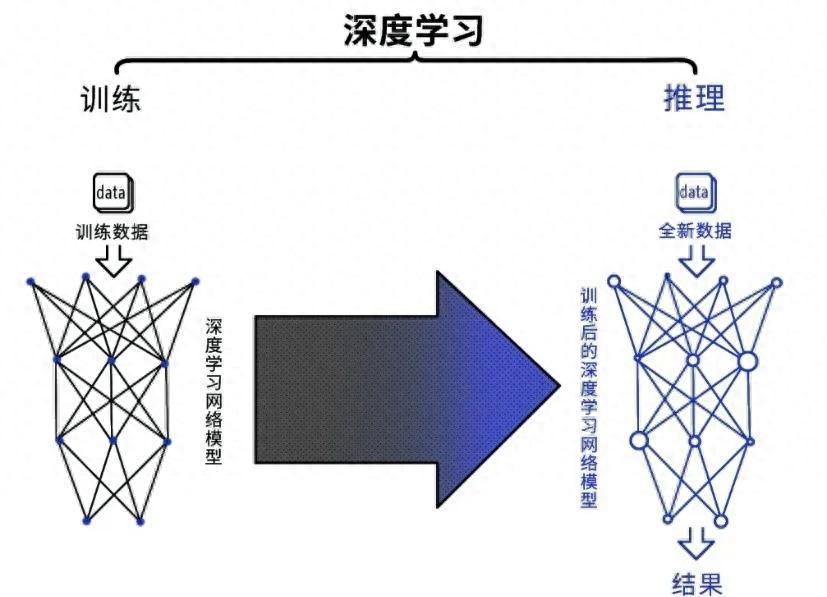
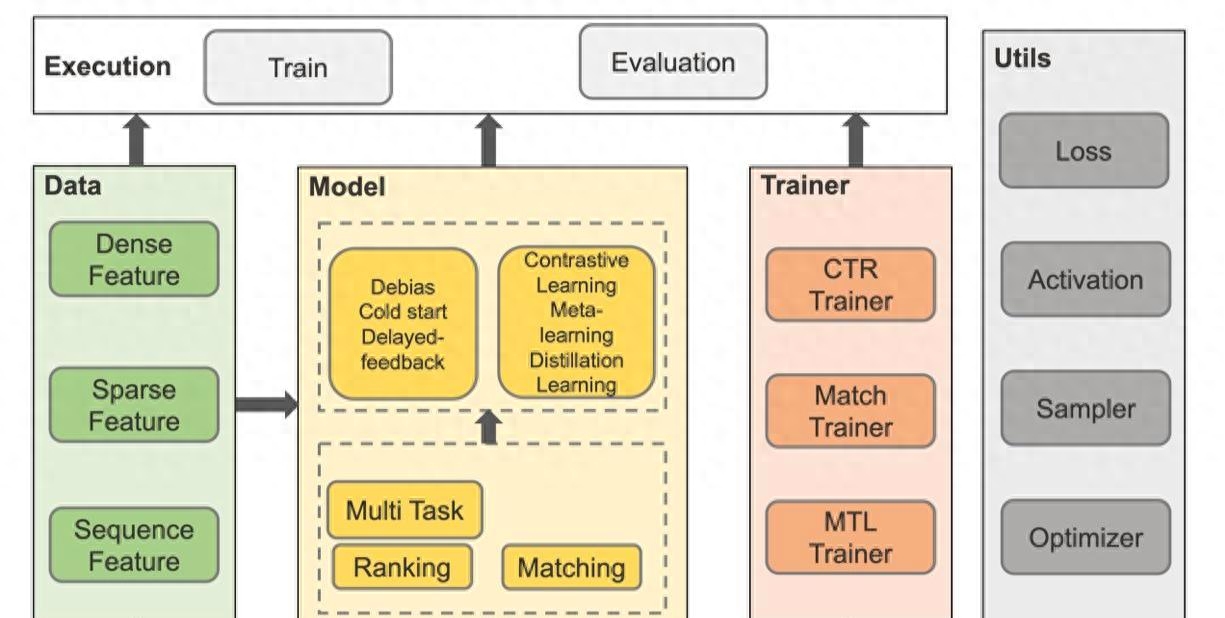
The above is the detailed content of The new frontier of personalized recommendations: the application of deep learning in recommendation systems. For more information, please follow other related articles on the PHP Chinese website!