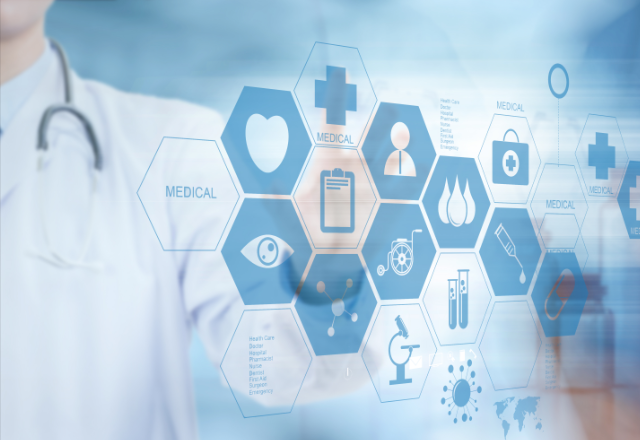
Generalist Medical AI (GMAI) models have the potential to revolutionize healthcare by providing advanced medical diagnostics, decision support and patient care capabilities.
The field of artificial intelligence (AI) has made significant progress in recent years, and fundamental models are at the forefront of this revolution. According to a new study published in the journal Nature, these powerful AI models, known as GMAI (General Medical Artificial Intelligence), have the potential to provide unprecedented improvements in medical diagnosis, decision support and patient care. capabilities to reshape healthcare. In this article, we take an in-depth look at a seminal study (see: https://www.nature.com/articles/s41586-023-05881-4) that outlines the GMAI model The transformative potential of AI and the challenges it poses is intended for professionals eager to explore the intersection of artificial intelligence and healthcare.
The power of base models
Foundation models are the latest generation of AI models that have been trained on a large number of different data sets, allowing them to perform well on a wide range of tasks . These models differ significantly from their predecessors, which were designed for one specific task at a time. With its versatility and state-of-the-art performance, the base model can answer questions, describe images, play video games and more. The advent of GMAI takes this versatility to new heights, promising to solve complex medical tasks and transform the healthcare landscape.
GMAI: Artificial Intelligence Transforming Healthcare
While medical AI has made great strides in specific task-oriented models, it has yet to embrace the power of underlying models. Traditional medical AI models are limited to predefined tasks, require extensive annotation efforts and lack adaptability to new tasks or data distributions. GMAI models, on the other hand, get rid of these limitations and offer three key features that set them apart from traditional medical AI models.
Flexible Interaction
GMAI models give users fine-grained control over their output, making complex medical information easier to access and understand. Users can customize output formats, rewrite responses, and even adjust the level of detail in specific fields. GMAI models can adapt to regional practices and communicate effectively with diverse users, transcending language barriers and adapting to personal preferences.
Adaptability
One of the distinguishing features of GMAI models is their ability to learn new tasks on the fly without extensive retraining. This contextual learning enables GMAI to keep up with emerging diseases, changing technologies, and evolving data distributions. By simply providing a few examples or hints, the GMAI model can quickly adapt to new scenarios, making it highly adaptable and future-proof.
Medical Domain Knowledge
GMAI models bring formal representations of medical knowledge to the table, enabling advanced medical reasoning and precise clinical interpretation. By leveraging knowledge graphs, retrieval-based methods, and existing medical databases, the GMAI model can reason through complex medical concepts and relationships. They can generate self-explanatory warnings, draft comprehensive radiology reports, provide detailed decision support at the bedside, and even generate protein sequences with desired properties.
Potential use cases for GMAI
The study highlights several exciting use cases where general medical AI models could have a profound impact on healthcare:
-
Grounded Radiology Reporting: The GMAI model can serve as a versatile digital radiology assistant, drafting comprehensive reports describing abnormalities, associated normal findings, and patient history. Through interactive visualizations, such as clickable links that highlight specific areas in images, radiologists can enhance their understanding and efficiency when interpreting complex cases.
-
Augmented Surgery: GMAI models can assist surgical teams by annotating live video streams, issuing alerts for missed steps, and providing relevant literature during surgery. By combining visual, linguistic, and anatomical knowledge, GMAI models can reason through complex surgical tasks and help surgeons navigate challenging scenarios.
-
Bedside Decision Support: The GMAI model can be used as a bedside clinical decision support tool, providing clinicians with detailed explanations, warnings and treatment recommendations based on real-time patient data. By summarizing complex electronic health records, predicting future patient status, and comparing treatment options, GMAI models can help clinicians make informed decisions and improve patient outcomes.
-
Interactive Recording: The GMAI model can revolutionize the documentation process by monitoring patient information and clinician-patient conversations and preemptively drafting electronic notes and discharge reports. This automation reduces administrative burden, allowing clinicians to allocate more time to direct patient care and improve overall workflow efficiency.
-
Patient Chatbots: GMAI is opening new avenues for patient support by enabling personalized chatbots that can deliver high-quality care outside of clinical settings. These chatbots can analyze a variety of patient data, from symptoms to monitoring readings, and provide detailed recommendations and explanations. The GMAI model makes healthcare more accessible, providing clear, easy-to-read information and reducing reliance on human experts to provide personalized support.
-
From text to protein generation: GMAI has the potential to revolutionize protein design by generating amino acid sequences and three-dimensional structures from textual prompts. This groundbreaking application could accelerate protein engineering, making it possible to design proteins with desired functional properties. GMAI's adaptability and ability to learn from a small number of examples make it a powerful tool in the fields of molecular biology and drug development.
Challenges and Considerations
While general medical AI models hold great promise, they also present unique challenges that must be addressed to ensure their safe and effective deployment in clinical settings.
-
Confirmation: The extreme versatility of GMAI models makes full validation a complex task. Validating a model against predefined use cases is relatively simple, but GMAI's ability to dynamically learn new tasks requires a proactive approach to testing and supervision. Clear guidelines and rigorous validation protocols must be established to ensure the accuracy and reliability of GMAI models in various medical scenarios.
-
Verification: The GMAI model has complex inputs and outputs, which brings challenges to verifying its correctness. Involvement of multidisciplinary teams and integration of interpretability techniques can aid the validation process. Providing clickable links to supporting passages in the literature can also enhance the fact-checking process and build trust in GMAI outputs.
-
Social Bias: Biases in artificial intelligence models have always been a problem, especially in health care, where they can perpetuate disparities and harm marginalized populations. GMAI models must be thoroughly audited to ensure they perform equally well in all types of patients. Ongoing monitoring and prompt action to mitigate bias are critical to ensuring fair and equitable health care outcomes.
-
Privacy: The development and use of GMAI models raise privacy concerns because they can access sensitive patient information. Strong privacy protocols, de-identification technology, and limiting the collection of individual patient data can help reduce privacy risks. Striking a balance between data accessibility and patient confidentiality is critical for responsible GMAI deployment.
-
Scale: GMAI models are computationally intensive and require large-scale data sets for training. The costs associated with data collection, model training, and computing resources are significant challenges. Collaborative efforts, data sharing initiatives, and responsible model optimization techniques are needed to overcome these barriers and ensure broad accessibility of GMAI models.
Healthcare Revolution
Generalist Medical AI models have the potential to revolutionize healthcare by delivering advanced medical diagnostics, decision support and patient care capabilities. Their flexibility, adaptability and integration of medical domain knowledge open up new possibilities for a variety of clinical applications. However, challenges related to validation, validation, bias, privacy, and scalability must be carefully addressed to ensure safe and ethical deployment of GMAI models.
As the AI community and clinical stakeholders continue to explore the potential of GMAI, it is critical to foster collaboration, establish regulatory frameworks, and prioritize patient-centered care. Through responsible development and widespread adoption, GMAI models can ease the burden.
The above is the detailed content of How does general medical artificial intelligence revolutionize the medical industry?. For more information, please follow other related articles on the PHP Chinese website!