經歷了提前兩天的「意外洩漏」之後,Llama 3.1 終於在昨夜由官方正式發布了。 Llama 3.1 將上下文長度擴展到了 128K,擁有 8B、70B 和 405B 三個版本,再次以一已之力抬高了大模型賽道的競爭標準。對 AI 社群來說,Llama 3.1 405B 最重要的意義是刷新了開源基礎模型的能力上限,Meta 官方稱,在一系列任務中,其性能可與最好的閉源模型相媲美。下表展示了目前 Llama 3 系列模型在關鍵基準測試上的表現。可以看出,405B 模型的表現與 GPT-4o 十分接近。
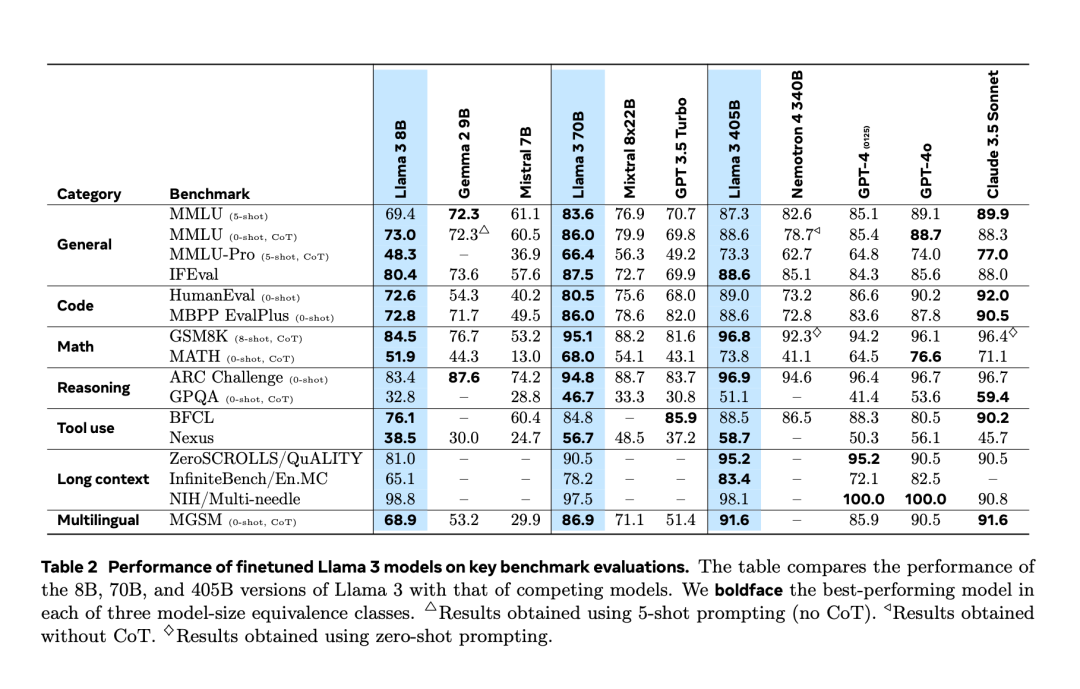
同時,Meta 公佈了《The Llama 3 Herd of Models》論文,揭示了 Llama 3 系列模型迄今為止的研究細節。
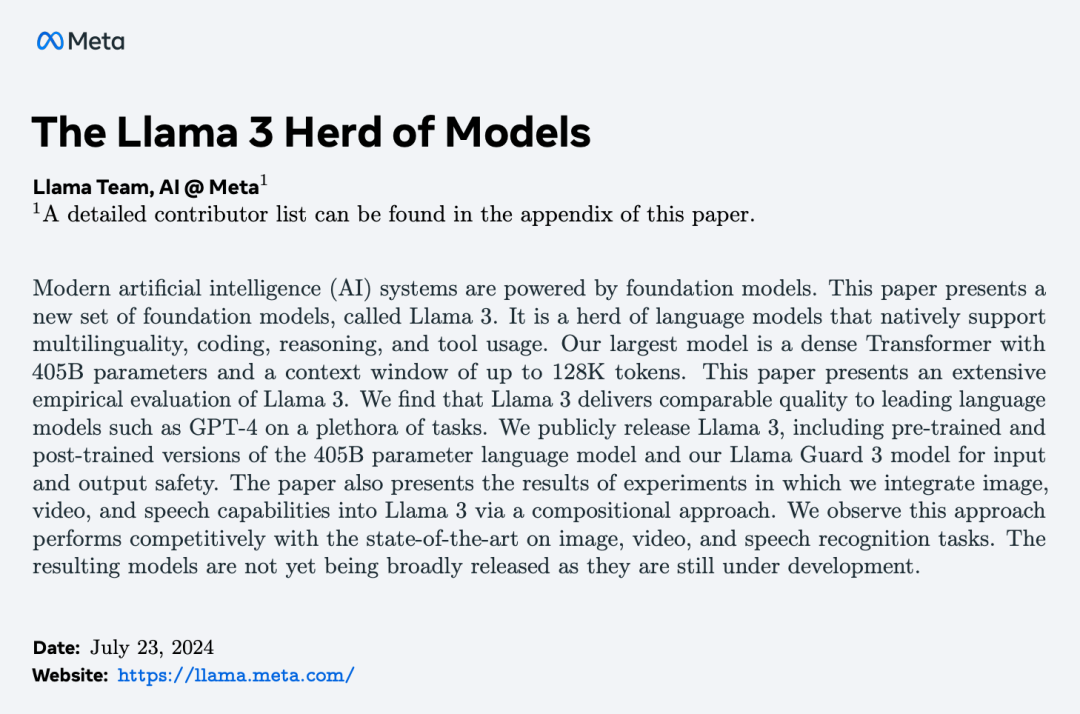
論文地址中:https://ai.meta.com/research/publications/the-llama-33-herd--https://ai.meta.com/research/publications/the-llama-33-herd-llama-333 3/L3330370370370373 月論文 -37337533333363
Llama 3.1 405B 在使用8K 上下文長度進行預訓練後,以128K 情境長度進行連續訓練,支援多語言和工具使用。 Meta 加強了 Llama 模型的預處理和預訓練資料的 Curation pipelines,以及後訓練資料的品質保證和過濾方法。
- Meta 認為,高品質基礎模型的開發有三個關鍵槓桿:資料、規模和複雜性管理。
-
資料:
與早期 Llama 版本相比,Meta 數量和品質兩方面改進了預訓練和後訓練資料。 Llama 3 在約 15 兆的多語言 Token 語料庫上進行預訓練,而 Llama 2 僅使用 1.8 兆 Token。
- 規模:訓練的模型規模遠大於先前的 Llama 模型:旗艦語言模型使用 3.8 x 10^25 次浮點運算(FLOPs)進行預訓練,超過 Llama 2 最大版本近 50 倍。
- 複雜性管理:根據 Scaling law,Meta 的旗艦模型已近似計算最優規模,但較小模型的訓練時間已遠超計算最優時長。結果表明,這些較小模型在相同推理預算下的表現優於計算最優模型。在後訓練階段,Meta 使用 405B 旗艦模型進一步提高了 70B 和 8B 等較小模型的品質。
- 為支援 405B 模型的大規模生產推理,Meta 將 16 位元 (BF16) 量化為 8 位元 (FP8),降低了計算要求,使模型能夠在單一伺服器節點上運行。
在 15.6T token(3.8x10^25 FLOPs)上預訓練 405B 是一項重大挑戰,Meta 優化了整個訓練堆疊,並使用了超過 16K H100 GPU。
- 正如 PyTorch 創始人、Meta 傑出工程師 Soumith Chintala 所說,Llama3 論文揭示了許多很酷的細節,其中之一就是基礎設施的構建。
- 1. 在訓練中,Meta 透過多輪對齊來完善 Chat 模型,其中包括監督微調(SFT)、拒絕取樣和直接偏好最佳化。大多數 SFT 樣本由合成資料產生。
- The researchers made several choices in the design to maximize the scalability of the model development process. For example, a standard dense Transformer model architecture was chosen with only minor adjustments rather than a mixture of experts to maximize training stability. Likewise, a relatively simple post-training procedure is adopted, based on supervised fine-tuning (SFT), rejection sampling (RS), and direct preference optimization (DPO), rather than more complex reinforcement learning algorithms, which tend to be less stable and more difficult Extension.
- As part of the Llama 3 development process, the Meta team also developed multi-modal extensions of the model, giving it capabilities in image recognition, video recognition, and speech understanding. These models are still under active development and not yet ready for release, but the paper presents the results of preliminary experiments with these multimodal models.
- Meta has updated its license to allow developers to use the output of Llama models to enhance other models.
- At the end of this paper, we also saw a long list of contributors: fenye1. This series of factors finally created the Llama 3 series today.
- Of course, for ordinary developers, how to utilize the 405B scale Llama model is a challenge and requires a lot of computing resources and expertise.
- After launch, Llama 3.1’s ecosystem is ready, with over 25 partners offering services that work with the latest model, including Amazon Cloud Technologies, NVIDIA, Databricks, Groq, Dell, Azure, Google Cloud, Snowflake, and more .
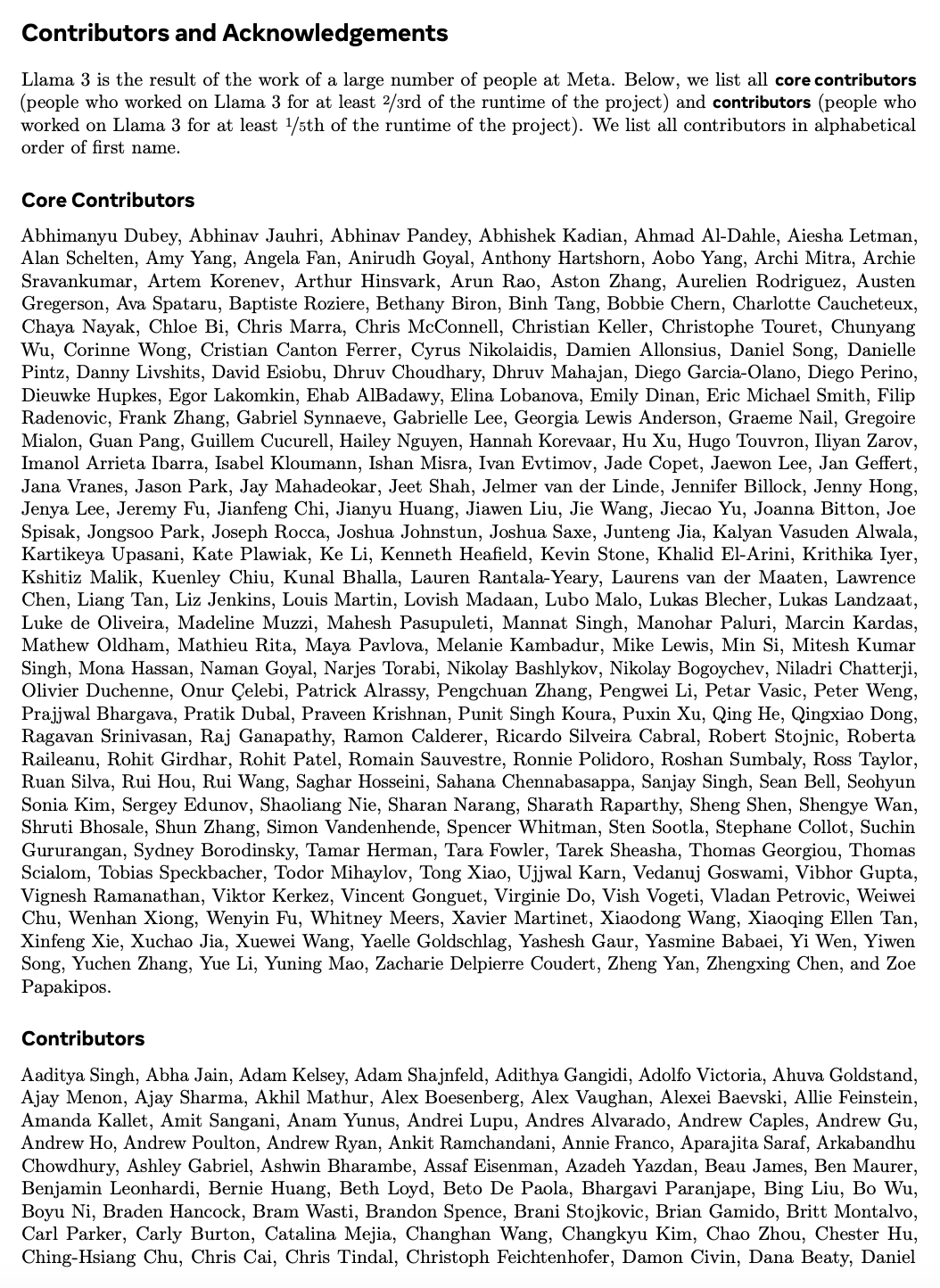
For more technical details, please refer to the original paper.
以上是擊敗GPT-4o的開源模型如何煉成?關於Llama 3.1 405B,Meta都寫在這篇論文裡了的詳細內容。更多資訊請關注PHP中文網其他相關文章!