


Vincent Tu's new SOTA! Pika, Peking University and Stanford jointly launch RPG, multi-modal to help solve two major problems of Wenshengtu
Recently, Peking University, Stanford, and the popular Pika Labs jointly published a study that takes the capabilities of large-model Vincentian graphs to a new level.
Paper address: https://arxiv.org/pdf/2401.11708.pdf
Code Address: https://github.com/YangLing0818/RPG-DiffusionMaster
The author of the paper proposed an innovative method, using the reasoning capabilities of the multi-modal large language model (MLLM), to improve the text-to-image generation/editing framework.
In other words, this method aims to improve the performance of text generation models when processing complex text prompts containing multiple attributes, relationships, and objects.
Without further ado, here’s the picture:
A green twintail girl in orange dress is sitting on the sofa while a messy desk under a big window on the left, a lively aquarium is on the top right of the sofa, realistic style.
A wearing orange dress girl with twin tails is sitting on the sofa, next to the big window is a messy desk, with a lively aquarium on the upper right, room style realism.
# Faced with multiple objects with complex relationships, the structure of the entire picture and the relationship between people and objects given by the model are very reasonable, making the viewer's eyes shine.
And for the same prompt, let’s take a look at the performance of the current state-of-the-art SDXL and DALL·E 3:
Let’s take a look at the performance of the new framework when binding multiple properties to multiple objects:
From left to right, a blonde ponytail European girl in white shirt, a brown curly hair African girl in blue shirt printed with a bird, an Asian young man with black short hair in suit are walking in the campus happily.
From left to right, a European girl wearing a white shirt with a blond ponytail, an African girl with brown curly hair wearing a blue shirt with a bird printed on it, and an Asian girl wearing a suit with short black hair. Young people are walking happily on campus.
The researchers named this framework RPG (Recaption, Plan and Generate), using MLLM as the global planner to decompose the complex image generation process into multiple sub-regions. A simpler build task.
The paper proposes complementary regional diffusion to achieve regional combination generation, and also integrates text-guided image generation and editing into the RPG framework in a closed-loop manner , thus enhancing the generalization ability.
Experiments show that the RPG framework proposed in this article is better than the current state-of-the-art text image diffusion models, including DALL·E 3 and SDXL, especially in multi-category object synthesis and text image semantics Alignment aspect.
It is worth noting that the RPG framework is widely compatible with various MLLM architectures (such as MiniGPT-4) and diffusion backbone networks (such as ControlNet).
RPG
#The current Vincentian graph model mainly has two problems: 1. Layout-based or attention-based methods can only provide rough spatial guidance and are difficult to Handle overlapping objects; 2. Feedback-based methods require collecting high-quality feedback data and incur additional training costs.
In order to solve these problems, researchers proposed three core strategies of RPG, as shown in the figure below:
Given a complex text prompt containing multiple entities and relationships, MLLM is first used to decompose it into basic prompts and highly descriptive sub-prompts; subsequently, the CoT planning of the multi-modal model is used to divide the image space into Complementary sub-regions; finally, complementary region diffusion is introduced to generate images of each sub-region independently and aggregate at each sampling step.
Multi-modal re-tuning
Convert textual cues into highly descriptive cues, providing information-enhanced cue understanding and semantic alignment in diffusion models.
Use MLLM to identify key phrases in user prompt y and obtain the sub-items:
# #Use LLM to decompose the text prompt into different sub-prompts and redescribe them in more detail:
In this way, you can Generate denser fine-grained details for each sub-cue to effectively increase the fidelity of the generated images and reduce the semantic differences between cues and images.
Thought chain planning
Divide the image space into complementary sub-regions and assign different sub-prompts while breaking down the build task into multiple simpler sub-tasks.
Specifically, the image space H × W is divided into several complementary regions, and each enhancer prompt is assigned to a specific region R:
Use MLLM’s powerful thinking chain reasoning capabilities to carry out effective regional division. By analyzing the retrieved intermediate results, detailed principles and precise instructions can be generated for subsequent image synthesis.
Supplementary Area Diffusion
In each rectangular sub-area, content guided by sub-cues is independently generated and subsequently resized and connected. , spatially merge these sub-regions.
This method effectively solves the problem of large models having difficulty processing overlapping objects. Furthermore, the paper extends this framework to adapt to editing tasks, employing contour-based region diffusion to precisely operate on inconsistent regions that need modification.
Text-guided image editing
As shown in the image above. In the retelling stage, RPG uses MLLM as subtitles to retell the source image, and uses its powerful reasoning capabilities to identify fine-grained semantic differences between the image and the target cue, directly analyzing how the input image aligns with the target cue.
Use MLLM (GPT-4, Gemini Pro, etc.) to check differences between input and target regarding numerical accuracy, property bindings, and object relationships. The resulting multimodal understanding feedback will be delivered to the MLLM for inferential editing planning.
Let’s take a look at the performance of the generation effect in the above three aspects. The first is attribute binding, comparing SDXL, DALL·E 3 and LMD:
We can see that across all three tests, only the RPG most accurately reflects what the prompts describe.
Then there is numerical accuracy, the display order is the same as above (SDXL, DALL·E 3, LMD, RPG):
——I didn’t expect that counting would be quite difficult for the large model of Vincent. The RPG easily defeated the opponent.
The last item is the complex relationship in the restore prompt:
In addition, you can also Diffusion expands into a hierarchical format, dividing a specific sub-region into smaller sub-regions.
As shown in the figure below, when adding a hierarchy of region segmentation, RPG can achieve significant improvements in text-to-image generation. This provides a new perspective for handling complex generation tasks, making it possible to generate images of arbitrary composition.
The above is the detailed content of Vincent Tu's new SOTA! Pika, Peking University and Stanford jointly launch RPG, multi-modal to help solve two major problems of Wenshengtu. For more information, please follow other related articles on the PHP Chinese website!

Hot AI Tools

Undresser.AI Undress
AI-powered app for creating realistic nude photos

AI Clothes Remover
Online AI tool for removing clothes from photos.

Undress AI Tool
Undress images for free

Clothoff.io
AI clothes remover

AI Hentai Generator
Generate AI Hentai for free.

Hot Article

Hot Tools

Notepad++7.3.1
Easy-to-use and free code editor

SublimeText3 Chinese version
Chinese version, very easy to use

Zend Studio 13.0.1
Powerful PHP integrated development environment

Dreamweaver CS6
Visual web development tools

SublimeText3 Mac version
God-level code editing software (SublimeText3)

Hot Topics
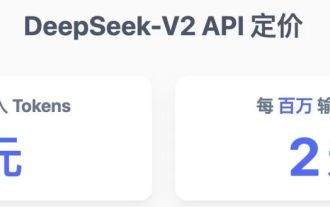
Imagine an artificial intelligence model that not only has the ability to surpass traditional computing, but also achieves more efficient performance at a lower cost. This is not science fiction, DeepSeek-V2[1], the world’s most powerful open source MoE model is here. DeepSeek-V2 is a powerful mixture of experts (MoE) language model with the characteristics of economical training and efficient inference. It consists of 236B parameters, 21B of which are used to activate each marker. Compared with DeepSeek67B, DeepSeek-V2 has stronger performance, while saving 42.5% of training costs, reducing KV cache by 93.3%, and increasing the maximum generation throughput to 5.76 times. DeepSeek is a company exploring general artificial intelligence
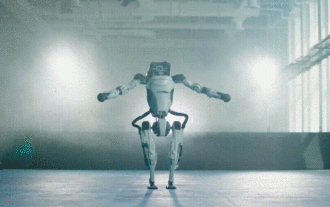
Boston Dynamics Atlas officially enters the era of electric robots! Yesterday, the hydraulic Atlas just "tearfully" withdrew from the stage of history. Today, Boston Dynamics announced that the electric Atlas is on the job. It seems that in the field of commercial humanoid robots, Boston Dynamics is determined to compete with Tesla. After the new video was released, it had already been viewed by more than one million people in just ten hours. The old people leave and new roles appear. This is a historical necessity. There is no doubt that this year is the explosive year of humanoid robots. Netizens commented: The advancement of robots has made this year's opening ceremony look like a human, and the degree of freedom is far greater than that of humans. But is this really not a horror movie? At the beginning of the video, Atlas is lying calmly on the ground, seemingly on his back. What follows is jaw-dropping
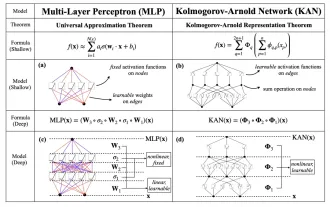
Earlier this month, researchers from MIT and other institutions proposed a very promising alternative to MLP - KAN. KAN outperforms MLP in terms of accuracy and interpretability. And it can outperform MLP running with a larger number of parameters with a very small number of parameters. For example, the authors stated that they used KAN to reproduce DeepMind's results with a smaller network and a higher degree of automation. Specifically, DeepMind's MLP has about 300,000 parameters, while KAN only has about 200 parameters. KAN has a strong mathematical foundation like MLP. MLP is based on the universal approximation theorem, while KAN is based on the Kolmogorov-Arnold representation theorem. As shown in the figure below, KAN has
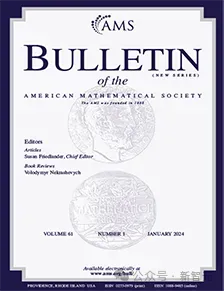
AI is indeed changing mathematics. Recently, Tao Zhexuan, who has been paying close attention to this issue, forwarded the latest issue of "Bulletin of the American Mathematical Society" (Bulletin of the American Mathematical Society). Focusing on the topic "Will machines change mathematics?", many mathematicians expressed their opinions. The whole process was full of sparks, hardcore and exciting. The author has a strong lineup, including Fields Medal winner Akshay Venkatesh, Chinese mathematician Zheng Lejun, NYU computer scientist Ernest Davis and many other well-known scholars in the industry. The world of AI has changed dramatically. You know, many of these articles were submitted a year ago.
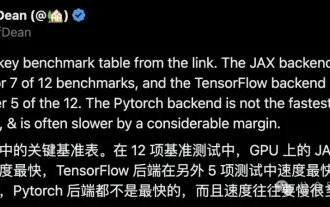
The performance of JAX, promoted by Google, has surpassed that of Pytorch and TensorFlow in recent benchmark tests, ranking first in 7 indicators. And the test was not done on the TPU with the best JAX performance. Although among developers, Pytorch is still more popular than Tensorflow. But in the future, perhaps more large models will be trained and run based on the JAX platform. Models Recently, the Keras team benchmarked three backends (TensorFlow, JAX, PyTorch) with the native PyTorch implementation and Keras2 with TensorFlow. First, they select a set of mainstream
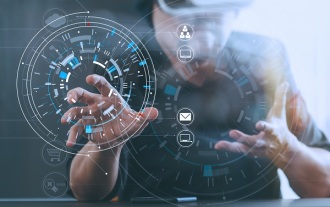
Face detection and recognition technology is already a relatively mature and widely used technology. Currently, the most widely used Internet application language is JS. Implementing face detection and recognition on the Web front-end has advantages and disadvantages compared to back-end face recognition. Advantages include reducing network interaction and real-time recognition, which greatly shortens user waiting time and improves user experience; disadvantages include: being limited by model size, the accuracy is also limited. How to use js to implement face detection on the web? In order to implement face recognition on the Web, you need to be familiar with related programming languages and technologies, such as JavaScript, HTML, CSS, WebRTC, etc. At the same time, you also need to master relevant computer vision and artificial intelligence technologies. It is worth noting that due to the design of the Web side
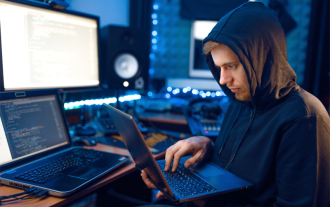
Let me introduce to you the latest AIGC open source project-AnimagineXL3.1. This project is the latest iteration of the anime-themed text-to-image model, aiming to provide users with a more optimized and powerful anime image generation experience. In AnimagineXL3.1, the development team focused on optimizing several key aspects to ensure that the model reaches new heights in performance and functionality. First, they expanded the training data to include not only game character data from previous versions, but also data from many other well-known anime series into the training set. This move enriches the model's knowledge base, allowing it to more fully understand various anime styles and characters. AnimagineXL3.1 introduces a new set of special tags and aesthetics
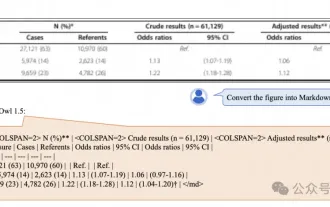
New SOTA for multimodal document understanding capabilities! Alibaba's mPLUG team released the latest open source work mPLUG-DocOwl1.5, which proposed a series of solutions to address the four major challenges of high-resolution image text recognition, general document structure understanding, instruction following, and introduction of external knowledge. Without further ado, let’s look at the effects first. One-click recognition and conversion of charts with complex structures into Markdown format: Charts of different styles are available: More detailed text recognition and positioning can also be easily handled: Detailed explanations of document understanding can also be given: You know, "Document Understanding" is currently An important scenario for the implementation of large language models. There are many products on the market to assist document reading. Some of them mainly use OCR systems for text recognition and cooperate with LLM for text processing.
