


Human-mouse hybrid brain appears! Stanford University 7-year research published in Nature
This article is reprinted with the authorization of AI New Media Qubit (public account ID: QbitAI). Please contact the source for reprinting.
Brain-like organoids from humans have now been successfully combined with mouse brains in the hands of scientists.
And if you touch a mouse's whisker, these brain cells can react normally.
The results of this study from Stanford were published in the latest issue of Nature.
How deep is this "human-mouse hybrid brain" combination?
In the own words of the corresponding author, Stanford neuroscientist Sergiu Pasca :
It is like adding a new transistor to the circuit. What the hell does this happen? Integrating human brain-like tissue into the rat brainTo make this clear, we have to start withorganoids.
Organoids are a collection of cells cultured from human stem cells such as embryonic stem cells and induced pluripotent stem cells. In recent years, organoids have been a major academic hot spot in the field of neuroscience because these three-dimensional micro-organs contain some key characteristics of their representative organs and can be used to simulate human development and disease in vitro. In other words, with the help of organoids, scientists can directly observe various structures of the kidneys, intestines, and even the brain in a petri dish to explore the underlying neural mechanisms. Convenience is convenience, but organoids also have many limitations. For example, although brain organoids can imitate the human brain, they are difficult to establish neural connections like a real brain, and cannot be integrated with other neural circuits that control behavior. Researchers at Stanford University aim to break through this limitation. Their experimental plan is: First, use human stem cells to cultivate a brain-like structure similar to the cerebral cortex. Then, it was transplanted into the somatosensory cortex of the brain of athymic rats that were 2-3 days old. This area of the rat's brain receives signals from the whiskers and other sensory organs and passes them on to other brain areas.become part of it!
By this time, the researchers found that human cells in the somatosensory cortex of mice also responded when they touched their whiskers. In addition, the researchers also specially processed some human brain tissue so that individual neurons can be activated by blue light of specific frequencies. The rats implanted with these human brain tissues participated in a new training: ultra-thin optical fibers were implanted in their brains. When these optical fibers emitted blue light, they could drink from the tap. to the water. The results showed that the rats learned to associate blue light with drinking water. This showed that the implanted human brain cells were actually involved in the work of the rat brain. It is worth noting that the researchers mentioned that brain organoids transplanted into rat brains are more complex in form and function than brain organoids cultured in vitro.further explore human neurodegenerative diseases and mental illnesses, etc., and then develop some effective new drugs. The epilepsy, Alzheimer's disease, Parkinson's syndrome, and amyotrophic lateral sclerosis that we often hear about are all neurodegenerative diseases.
In order to gain a deeper understanding of brain tissue activity in patients with neurological-related diseases, this research lasted for 7 years.
In one experiment, researchers implanted brain organoids grown from stem cells of patients with Timothy Syndrome (TS) into a part of the brain of mice. side, and another time implanted into a healthy human brain organoid for comparison.
TiMosey syndrome is a rare genetic disease, and patients are prone to arrhythmia)Five to six months after implantation in mice, they observed that the diseased cells were smaller and engaged in electrical activity that was completely different from healthy brain cells.
Moreover, the dendrite morphology at the terminals of TS neurons has been significantly changed.
Further analysis found that at a similar differentiation stage, the dendritic branching pattern of TS t-hCO in rat brains was abnormal, while the dendritic branching of TS t-hCO cultured in vitro did not show this pattern. situation.
The researchers noted that the branching patterns in the rats' brains were abnormal, consistent with their previous research on the disease.
It can be seen that the effect of revealing disease phenotypes by implanting human brains into rats is quite good.
Overall, this research has attracted the attention of many people in the biological and medical circles.
Madeline A. Lancaster, member of the Medical Research Council of Cambridge, UK
commented:The study is overall quite progressive. , providing a new way to understand diseases involving brain cell dysfunction.
But in fact, it seems that no major problems have occurred so far, and the mice have not become smarter due to the implantation of human-like brains. A report released last year by the National Academies of Sciences, Engineering and Medicine stated: "Brain organoids derived from humans are still too primitive to form consciousness or human intelligence." This article Corresponding author Professor Pascal said that judging from their experimental process and results, rats tolerated human brain organoids very well, and the transplantation did not cause problems such as epilepsy. Despite this, there are still many skeptical voices in the academic community. Arlotta, a member of the National Academy of Sciences panel, held a different view. She felt that with the development of science, new problems are likely to arise. She said that the topic of "the integration of human organs with other organisms" should be continuously paid attention to and must not be discussed just once and then ignored. Especially for primates such as monkeys and orangutans, they are more similar to humans, so human brain-like organs are likely to develop more maturely in their bodies and have a greater impact on animal behavior. In this regard, Professor Pascal said that they will not conduct such research in the future and do not encourage others to conduct such research. In addition, some researchers pointed out the limitations of this study: Although the results of implanting the "mini human brain" into rats were better than other previous animal experiments, Due to spatiotemporal and cross-species limitations, highly reducible human neural circuits cannot be formed even when transplanted at early stages of development. Bennett Novitch, a member of UCLA’s Biomedical and Stem Cell Research Center, also commented: Using rats implanted with human brain tissue to test drugs is feasible for small studies, but it is not suitable for pharmaceuticals. Still not feasible for companies because of the need for speed and scale. Research TeamThe team that conducted this study came from the Department of Psychiatry and Behavioral Sciences and the Department of Comparative Medicine at Stanford University. Common act: Omer Revah, Felicity Gore, and Kevin W. Kelly. Omer Revah, Ph.D. in Physiology and Computational Neuroscience from Hebrew University. After graduating from his Ph.D., he worked as a postdoctoral researcher in the Department of Psychiatry and Behavioral Sciences at Stanford University. He is currently an assistant professor at the School of Veterinary Medicine at Hebrew University. Felicity Gore is currently engaged in research in the Department of Bioengineering at Stanford University. Kevin W. Kelly currently works in neuroscience and genomics in the Department of Psychiatry and Behavioral Sciences at Stanford. Corresponding author Sergiu PascaIt is worth noting that this research has also raised new ethical issues. The outside world is worried that these "human and other biological hybrids" will seriously damage animal welfare and even create "rat men".
is currently a professor in the Department of Psychiatry and Behavioral Sciences at Stanford University.
Paper address:
https://www.nature.com/articles/s41586-022-05277-w 参考链接:
[1]https://www.nature.com/articles/d41586-022-03238-x
[2]https://www.washingtonpost.com/science/2022/10/12/brain-tissue-rats-stanford/
The above is the detailed content of Human-mouse hybrid brain appears! Stanford University 7-year research published in Nature. For more information, please follow other related articles on the PHP Chinese website!

Hot AI Tools

Undresser.AI Undress
AI-powered app for creating realistic nude photos

AI Clothes Remover
Online AI tool for removing clothes from photos.

Undress AI Tool
Undress images for free

Clothoff.io
AI clothes remover

AI Hentai Generator
Generate AI Hentai for free.

Hot Article

Hot Tools

Notepad++7.3.1
Easy-to-use and free code editor

SublimeText3 Chinese version
Chinese version, very easy to use

Zend Studio 13.0.1
Powerful PHP integrated development environment

Dreamweaver CS6
Visual web development tools

SublimeText3 Mac version
God-level code editing software (SublimeText3)

Hot Topics
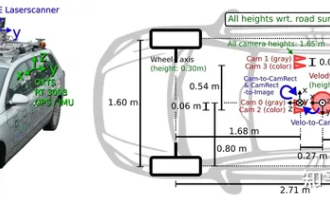
The first pilot and key article mainly introduces several commonly used coordinate systems in autonomous driving technology, and how to complete the correlation and conversion between them, and finally build a unified environment model. The focus here is to understand the conversion from vehicle to camera rigid body (external parameters), camera to image conversion (internal parameters), and image to pixel unit conversion. The conversion from 3D to 2D will have corresponding distortion, translation, etc. Key points: The vehicle coordinate system and the camera body coordinate system need to be rewritten: the plane coordinate system and the pixel coordinate system. Difficulty: image distortion must be considered. Both de-distortion and distortion addition are compensated on the image plane. 2. Introduction There are four vision systems in total. Coordinate system: pixel plane coordinate system (u, v), image coordinate system (x, y), camera coordinate system () and world coordinate system (). There is a relationship between each coordinate system,
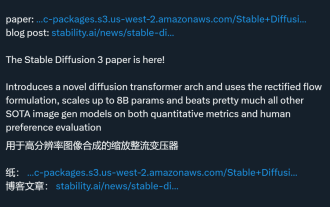
StableDiffusion3’s paper is finally here! This model was released two weeks ago and uses the same DiT (DiffusionTransformer) architecture as Sora. It caused quite a stir once it was released. Compared with the previous version, the quality of the images generated by StableDiffusion3 has been significantly improved. It now supports multi-theme prompts, and the text writing effect has also been improved, and garbled characters no longer appear. StabilityAI pointed out that StableDiffusion3 is a series of models with parameter sizes ranging from 800M to 8B. This parameter range means that the model can be run directly on many portable devices, significantly reducing the use of AI
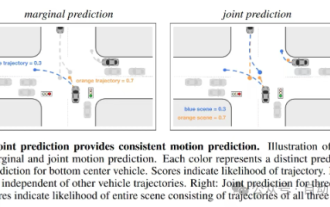
Trajectory prediction plays an important role in autonomous driving. Autonomous driving trajectory prediction refers to predicting the future driving trajectory of the vehicle by analyzing various data during the vehicle's driving process. As the core module of autonomous driving, the quality of trajectory prediction is crucial to downstream planning control. The trajectory prediction task has a rich technology stack and requires familiarity with autonomous driving dynamic/static perception, high-precision maps, lane lines, neural network architecture (CNN&GNN&Transformer) skills, etc. It is very difficult to get started! Many fans hope to get started with trajectory prediction as soon as possible and avoid pitfalls. Today I will take stock of some common problems and introductory learning methods for trajectory prediction! Introductory related knowledge 1. Are the preview papers in order? A: Look at the survey first, p
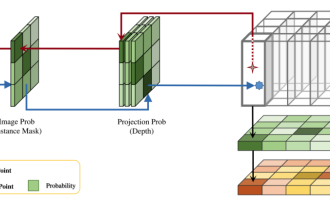
This paper explores the problem of accurately detecting objects from different viewing angles (such as perspective and bird's-eye view) in autonomous driving, especially how to effectively transform features from perspective (PV) to bird's-eye view (BEV) space. Transformation is implemented via the Visual Transformation (VT) module. Existing methods are broadly divided into two strategies: 2D to 3D and 3D to 2D conversion. 2D-to-3D methods improve dense 2D features by predicting depth probabilities, but the inherent uncertainty of depth predictions, especially in distant regions, may introduce inaccuracies. While 3D to 2D methods usually use 3D queries to sample 2D features and learn the attention weights of the correspondence between 3D and 2D features through a Transformer, which increases the computational and deployment time.
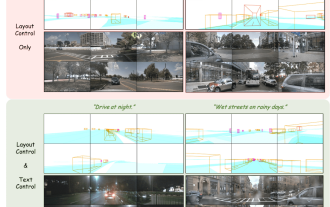
Some of the author’s personal thoughts In the field of autonomous driving, with the development of BEV-based sub-tasks/end-to-end solutions, high-quality multi-view training data and corresponding simulation scene construction have become increasingly important. In response to the pain points of current tasks, "high quality" can be decoupled into three aspects: long-tail scenarios in different dimensions: such as close-range vehicles in obstacle data and precise heading angles during car cutting, as well as lane line data. Scenes such as curves with different curvatures or ramps/mergings/mergings that are difficult to capture. These often rely on large amounts of data collection and complex data mining strategies, which are costly. 3D true value - highly consistent image: Current BEV data acquisition is often affected by errors in sensor installation/calibration, high-precision maps and the reconstruction algorithm itself. this led me to
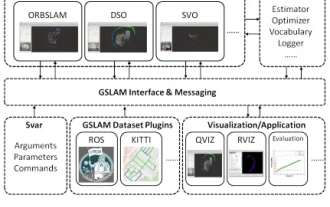
Suddenly discovered a 19-year-old paper GSLAM: A General SLAM Framework and Benchmark open source code: https://github.com/zdzhaoyong/GSLAM Go directly to the full text and feel the quality of this work ~ 1 Abstract SLAM technology has achieved many successes recently and attracted many attracted the attention of high-tech companies. However, how to effectively perform benchmarks on speed, robustness, and portability with interfaces to existing or emerging algorithms remains a problem. In this paper, a new SLAM platform called GSLAM is proposed, which not only provides evaluation capabilities but also provides researchers with a useful way to quickly develop their own SLAM systems.
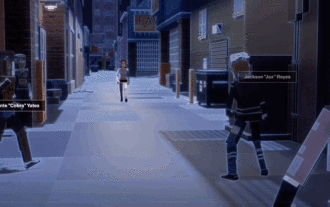
Please note that this square man is frowning, thinking about the identities of the "uninvited guests" in front of him. It turned out that she was in a dangerous situation, and once she realized this, she quickly began a mental search to find a strategy to solve the problem. Ultimately, she decided to flee the scene and then seek help as quickly as possible and take immediate action. At the same time, the person on the opposite side was thinking the same thing as her... There was such a scene in "Minecraft" where all the characters were controlled by artificial intelligence. Each of them has a unique identity setting. For example, the girl mentioned before is a 17-year-old but smart and brave courier. They have the ability to remember and think, and live like humans in this small town set in Minecraft. What drives them is a brand new,
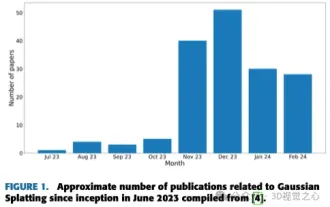
Written above & The author’s personal understanding is that image-based 3D reconstruction is a challenging task that involves inferring the 3D shape of an object or scene from a set of input images. Learning-based methods have attracted attention for their ability to directly estimate 3D shapes. This review paper focuses on state-of-the-art 3D reconstruction techniques, including generating novel, unseen views. An overview of recent developments in Gaussian splash methods is provided, including input types, model structures, output representations, and training strategies. Unresolved challenges and future directions are also discussed. Given the rapid progress in this field and the numerous opportunities to enhance 3D reconstruction methods, a thorough examination of the algorithm seems crucial. Therefore, this study provides a comprehensive overview of recent advances in Gaussian scattering. (Swipe your thumb up
