A Beginner's Guide to Visualizing Data with Python for EDA
Introduction
Data visualization is an essential part of Exploratory Data Analysis (EDA). EDA involves examining datasets to uncover patterns, detect anomalies, and understand relationships between variables. Visualization tools help present data insights in a clear and interpretable manner, allowing analysts to make data-driven decisions efficiently. Python, with its vast library ecosystem, has become the go-to programming language for EDA.
In this article, we’ll walk you through how to visualize data using Python for EDA. Whether you're a beginner or someone looking to refine your skills, this guide will cover the essential tools, libraries, and techniques.
1. Why Data Visualization Matters in EDA?
EDA helps analysts understand datasets by identifying patterns, trends, and anomalies.
Visualizing data offers several benefits:
Quick Interpretation: Graphs and plots make it easier to understand complex datasets.
Pattern Identification: Helps reveal correlations, trends, and outliers.
Data Quality Check: Visualization tools detect missing or erroneous values.
Better Communication: Visuals are an effective way to present findings to stakeholders.
2. Python Libraries for Data Visualization
Python offers several powerful libraries for visualizing data. Here are the key ones you’ll use during EDA:
2.1 Matplotlib
Matplotlib is the most fundamental plotting library in Python, providing tools to create static, animated, and interactive visualizations.
Best Use Case: Line charts, bar plots, and pie charts.
import matplotlib.pyplot as plt
x = [1, 2, 3, 4]
y = [10, 20, 25, 30]
plt.plot(x, y)
plt.title("Basic Line Plot")
plt.show()
2.2 Seaborn
Seaborn is built on top of Matplotlib and offers beautiful default styles, especially for statistical visualizations.
Best Use Case: Heatmaps, pair plots, and distribution plots.
import seaborn as sns
data = sns.load_dataset('iris')
sns.pairplot(data, hue='species')
plt.show()
2.3 Pandas Visualization
Pandas allows quick plotting directly from dataframes using df.plot(). It is perfect for beginners who want to get started with simple visualizations.
import pandas as pd
df = pd.DataFrame({'A': [1, 2, 3], 'B': [3, 2, 1]})
df.plot(kind='bar')
plt.show()
2.4 Plotly
Plotly is an interactive plotting library, suitable for creating dashboards and detailed visualizations.
Best Use Case: Interactive graphs that allow zooming and filtering.
import plotly.express as px
fig = px.scatter(x=[1, 2, 3], y=[3, 1, 6], title="Interactive Scatter Plot")
fig.show()
3. Types of Data Visualizations for EDA
Different types of visualizations serve different purposes in EDA. Below are the most common plot types and when to use them:
3.1 Line Plot
Use Case: Visualizing trends over time or continuous variables.
Library Example: Matplotlib.
import numpy as np
x = np.linspace(0, 10, 100)
y = np.sin(x)
plt.plot(x, y)
plt.title("Sine Wave Plot")
plt.show()
3.2 Bar Plot
Use Case: Comparing categorical data or frequency distributions.
Library Example: Seaborn.
python
Copy code
sns.countplot(x='species', data=data)
plt.show()
3.3 Histogram
Use Case: Understanding the distribution of a variable.
Library Example: Matplotlib, Seaborn.
sns.histplot(data['sepal_length'], bins=20, kde=True)
plt.show()
3.4 Scatter Plot
Use Case: Identifying relationships between two variables.
Library Example: Plotly, Seaborn.
sns.scatterplot(x='sepal_length', y='sepal_width', hue='species', data=data)
plt.show()
3.5 Heatmap
Use Case: Visualizing correlations between variables.
Library Example: Seaborn.
corr = data.corr()
sns.heatmap(corr, annot=True, cmap='coolwarm')
plt.show()
4. Practical Example: EDA on a Sample Dataset
Let’s apply our visualization techniques to a real dataset. For this example, we’ll use the Iris dataset to explore relationships between features.
Step 1: Load the Dataset
import seaborn as sns
import pandas as pd
data = sns.load_dataset('iris')
print(data.head())
Step 2: Create Pair Plots to Explore Relationships
sns.pairplot(data, hue='species')
plt.show()
This pair plot helps us visualize how features like sepal length and petal width are distributed across different species.
Step 3: Check for Missing Values with a Heatmap
sns.heatmap(data.isnull(), cbar=False, cmap='viridis')
plt.title("Missing Values Heatmap")
plt.show()
5. Handling Outliers with Visualizations
Detecting outliers is crucial during EDA to ensure model accuracy. Here’s how to spot outliers visually:
5.1 Box Plot for Outlier Detection
sns.boxplot(x='species', y='sepal_length', data=data)
plt.show()
In this box plot, outliers are shown as individual points beyond the whiskers.
6. Tips for Effective Data Visualization
Choose the Right Chart Type: Select visualizations that align with your data type (e.g., line plots for trends, bar plots for categorical data).
Use Color Wisely: Colors should add meaning; avoid excessive use of colors that can confuse readers.
Label Your Axes: Always add titles, axis labels, and legends to make plots interpretable.
Experiment with Interactivity: Use Plotly to create interactive dashboards for deeper insights.
Keep It Simple: Avoid cluttered visuals—focus on key insights.
7. Conclusion
Python offers a rich ecosystem of libraries for data visualization, making it an essential tool for exploratory data analysis (EDA). From Matplotlib and Seaborn for static plots to Plotly for interactive dashboards, Python caters to every need during EDA.
Visualizing data is not just about creating attractive plots—it’s about extracting meaningful insights and communicating them effectively. Whether you’re a beginner or an experienced analyst, mastering these visualization techniques will enhance your data analysis skills.
For further reading on exploratory data analysis techniques, explore this comprehensive guide here.
Keep experimenting with Python, and you’ll be uncovering valuable insights in no time!
The above is the detailed content of A Beginner's Guide to Visualizing Data with Python for EDA. For more information, please follow other related articles on the PHP Chinese website!

Hot AI Tools

Undress AI Tool
Undress images for free

Undresser.AI Undress
AI-powered app for creating realistic nude photos

AI Clothes Remover
Online AI tool for removing clothes from photos.

Clothoff.io
AI clothes remover

Video Face Swap
Swap faces in any video effortlessly with our completely free AI face swap tool!

Hot Article

Hot Tools

Notepad++7.3.1
Easy-to-use and free code editor

SublimeText3 Chinese version
Chinese version, very easy to use

Zend Studio 13.0.1
Powerful PHP integrated development environment

Dreamweaver CS6
Visual web development tools

SublimeText3 Mac version
God-level code editing software (SublimeText3)
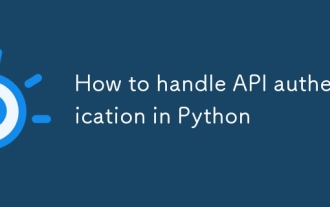
The key to dealing with API authentication is to understand and use the authentication method correctly. 1. APIKey is the simplest authentication method, usually placed in the request header or URL parameters; 2. BasicAuth uses username and password for Base64 encoding transmission, which is suitable for internal systems; 3. OAuth2 needs to obtain the token first through client_id and client_secret, and then bring the BearerToken in the request header; 4. In order to deal with the token expiration, the token management class can be encapsulated and automatically refreshed the token; in short, selecting the appropriate method according to the document and safely storing the key information is the key.
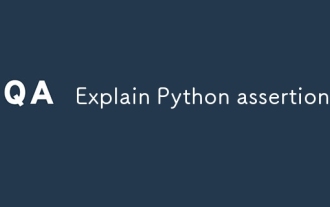
Assert is an assertion tool used in Python for debugging, and throws an AssertionError when the condition is not met. Its syntax is assert condition plus optional error information, which is suitable for internal logic verification such as parameter checking, status confirmation, etc., but cannot be used for security or user input checking, and should be used in conjunction with clear prompt information. It is only available for auxiliary debugging in the development stage rather than substituting exception handling.
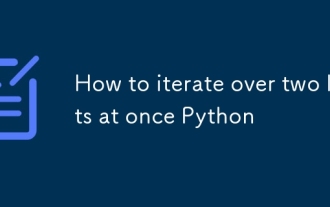
A common method to traverse two lists simultaneously in Python is to use the zip() function, which will pair multiple lists in order and be the shortest; if the list length is inconsistent, you can use itertools.zip_longest() to be the longest and fill in the missing values; combined with enumerate(), you can get the index at the same time. 1.zip() is concise and practical, suitable for paired data iteration; 2.zip_longest() can fill in the default value when dealing with inconsistent lengths; 3.enumerate(zip()) can obtain indexes during traversal, meeting the needs of a variety of complex scenarios.
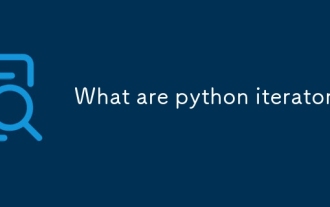
InPython,iteratorsareobjectsthatallowloopingthroughcollectionsbyimplementing__iter__()and__next__().1)Iteratorsworkviatheiteratorprotocol,using__iter__()toreturntheiteratorand__next__()toretrievethenextitemuntilStopIterationisraised.2)Aniterable(like
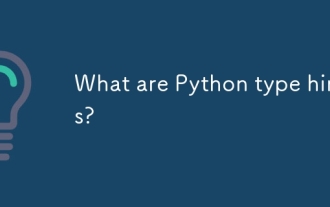
TypehintsinPythonsolvetheproblemofambiguityandpotentialbugsindynamicallytypedcodebyallowingdeveloperstospecifyexpectedtypes.Theyenhancereadability,enableearlybugdetection,andimprovetoolingsupport.Typehintsareaddedusingacolon(:)forvariablesandparamete
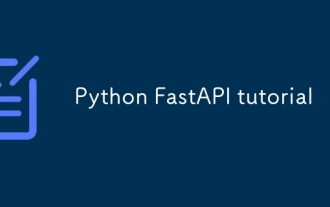
To create modern and efficient APIs using Python, FastAPI is recommended; it is based on standard Python type prompts and can automatically generate documents, with excellent performance. After installing FastAPI and ASGI server uvicorn, you can write interface code. By defining routes, writing processing functions, and returning data, APIs can be quickly built. FastAPI supports a variety of HTTP methods and provides automatically generated SwaggerUI and ReDoc documentation systems. URL parameters can be captured through path definition, while query parameters can be implemented by setting default values for function parameters. The rational use of Pydantic models can help improve development efficiency and accuracy.
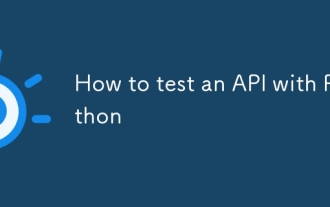
To test the API, you need to use Python's Requests library. The steps are to install the library, send requests, verify responses, set timeouts and retry. First, install the library through pipinstallrequests; then use requests.get() or requests.post() and other methods to send GET or POST requests; then check response.status_code and response.json() to ensure that the return result is in compliance with expectations; finally, add timeout parameters to set the timeout time, and combine the retrying library to achieve automatic retry to enhance stability.
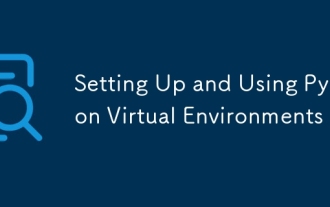
A virtual environment can isolate the dependencies of different projects. Created using Python's own venv module, the command is python-mvenvenv; activation method: Windows uses env\Scripts\activate, macOS/Linux uses sourceenv/bin/activate; installation package uses pipinstall, use pipfreeze>requirements.txt to generate requirements files, and use pipinstall-rrequirements.txt to restore the environment; precautions include not submitting to Git, reactivate each time the new terminal is opened, and automatic identification and switching can be used by IDE.
