


Implementing Machine Learning Algorithms in C++: Security Considerations and Best Practices
When implementing machine learning algorithms in C++, security considerations are critical, including data privacy, model tampering, and input validation. Best practices include adopting secure libraries, minimizing permissions, using sandboxes, and continuous monitoring. Practical examples demonstrate the use of the Botan library to encrypt and decrypt CNN models to ensure secure training and prediction.
Implementing Machine Learning Algorithms in C++: Security Considerations and Best Practices
Introduction
The security of machine learning algorithms is crucial, especially when dealing with sensitive data. This article discusses security considerations and best practices when implementing machine learning algorithms in C++.
Security Considerations
- Data Privacy: Ensure that the algorithm cannot access unauthorized data. Protect sensitive data using encryption such as AES or ChaCha20.
- Model tampering: Prevent malicious users from modifying the model to affect predictions. Use digital signatures or hashes to verify model integrity.
- Input validation: Validate input data to prevent injection attacks and data manipulation. Use data type validation, range checking, and regular expressions.
-
Memory Safety: Prevents buffer overflows and uninitialized variables that may cause algorithms to behave erratically. Use strict compiler flags (such as
-Weverything
) and follow safe coding practices.
Best Practices
- # Use secure libraries: Use audited and tested secure libraries such as Botan and Crypto++ , for encryption, hashing, and random number generation.
- Minimize permissions: Grant the minimum permissions required for algorithm execution and avoid using privileged accounts.
- Use sandboxing: Execute algorithms in a restricted environment to prevent them from accessing sensitive resources.
- Continuous monitoring: Monitor the security of algorithm deployments, looking for suspicious activity or patterns.
Practical case
Implementing a convolutional neural network (CNN) model for image classification while considering security:
#include <botan/botan.h> class SecureCNN { public: void train(const vector<Image>& images, const vector<Label>& labels) { // 加密图像和标签数据 Botan::Cipher_Block cipher("AES-256"); cipher.set_key("super secret key"); vector<EncryptedImage> encrypted_images; vector<EncryptedLabel> encrypted_labels; for (const auto& image : images) { encrypted_images.push_back(cipher.process(image)); } for (const auto& label : labels) { encrypted_labels.push_back(cipher.process(label)); } // 训练加密后的模型 EncryptedModel model; model.train(encrypted_images, encrypted_labels); // 保存加密后的模型 model.save("encrypted_model.bin"); } void predict(const Image& image) { // 加密图像数据 Botan::Cipher_Block cipher("AES-256"); cipher.set_key("super secret key"); EncryptedImage encrypted_image = cipher.process(image); // 使用加密后的模型进行预测 EncryptedLabel encrypted_label; encrypted_label = model.predict(encrypted_image); // 解密预测标签 Botan::Cipher_Block decipher("AES-256"); decipher.set_key("super secret key"); Label label = decipher.process(encrypted_label); return label; } };
Conclusion
The above is a guide to security considerations and best practices when using C++ to implement machine learning algorithms. By following these principles, you can help ensure the security of your algorithms and prevent data leaks and malicious tampering.
The above is the detailed content of Implementing Machine Learning Algorithms in C++: Security Considerations and Best Practices. For more information, please follow other related articles on the PHP Chinese website!

Hot AI Tools

Undresser.AI Undress
AI-powered app for creating realistic nude photos

AI Clothes Remover
Online AI tool for removing clothes from photos.

Undress AI Tool
Undress images for free

Clothoff.io
AI clothes remover

AI Hentai Generator
Generate AI Hentai for free.

Hot Article

Hot Tools

Notepad++7.3.1
Easy-to-use and free code editor

SublimeText3 Chinese version
Chinese version, very easy to use

Zend Studio 13.0.1
Powerful PHP integrated development environment

Dreamweaver CS6
Visual web development tools

SublimeText3 Mac version
God-level code editing software (SublimeText3)

Hot Topics
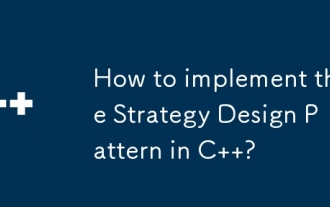
The steps to implement the strategy pattern in C++ are as follows: define the strategy interface and declare the methods that need to be executed. Create specific strategy classes, implement the interface respectively and provide different algorithms. Use a context class to hold a reference to a concrete strategy class and perform operations through it.
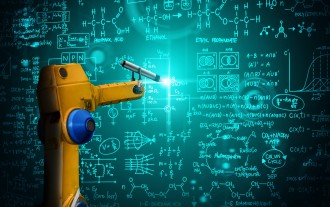
Machine learning is an important branch of artificial intelligence that gives computers the ability to learn from data and improve their capabilities without being explicitly programmed. Machine learning has a wide range of applications in various fields, from image recognition and natural language processing to recommendation systems and fraud detection, and it is changing the way we live. There are many different methods and theories in the field of machine learning, among which the five most influential methods are called the "Five Schools of Machine Learning". The five major schools are the symbolic school, the connectionist school, the evolutionary school, the Bayesian school and the analogy school. 1. Symbolism, also known as symbolism, emphasizes the use of symbols for logical reasoning and expression of knowledge. This school of thought believes that learning is a process of reverse deduction, through existing
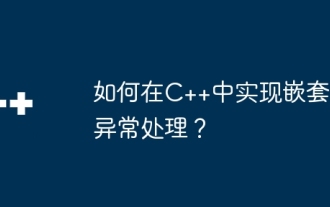
Nested exception handling is implemented in C++ through nested try-catch blocks, allowing new exceptions to be raised within the exception handler. The nested try-catch steps are as follows: 1. The outer try-catch block handles all exceptions, including those thrown by the inner exception handler. 2. The inner try-catch block handles specific types of exceptions, and if an out-of-scope exception occurs, control is given to the external exception handler.
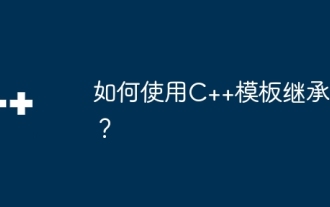
C++ template inheritance allows template-derived classes to reuse the code and functionality of the base class template, which is suitable for creating classes with the same core logic but different specific behaviors. The template inheritance syntax is: templateclassDerived:publicBase{}. Example: templateclassBase{};templateclassDerived:publicBase{};. Practical case: Created the derived class Derived, inherited the counting function of the base class Base, and added the printCount method to print the current count.
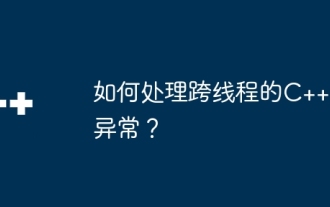
In multi-threaded C++, exception handling is implemented through the std::promise and std::future mechanisms: use the promise object to record the exception in the thread that throws the exception. Use a future object to check for exceptions in the thread that receives the exception. Practical cases show how to use promises and futures to catch and handle exceptions in different threads.
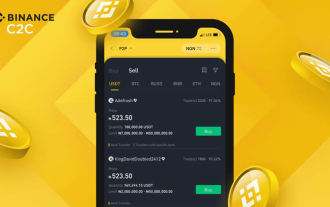
Binance C2C Trading Guide: Safe and convenient way to deposit and withdraw money in cryptocurrency. This article will explain the Binance C2C (CustomertoCustomer) trading model in detail, explain its security, characteristics and operation procedures, and provide graphic tutorials to help you easily master the Binance C2C deposit and withdraw money in ease. What is Binance C2C? Binance C2C is a user-to-user cryptocurrency trading service provided by the Binance platform, providing users with convenient cryptocurrency and fiat currency exchange channels. Launched in 2019, the service supports multiple cryptocurrencies and fiat currency transactions through a peer-to-peer trading model, and provides enhanced security and multiple features. Compared with traditional OTC trading, Binance C2C platform authenticates both parties to the transaction and provides complete support.
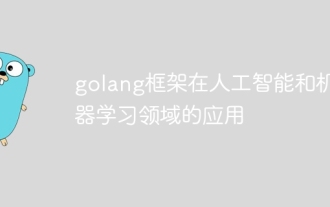
The Go framework has wide applications in the fields of artificial intelligence (AI) and machine learning (ML): TensorFlow provides GoAPI for building and training ML models. Keras provides a high-level neural network API for building and training deep learning models. GoAI is an AI framework written in Go that provides machine learning, neural network and computer vision modules.
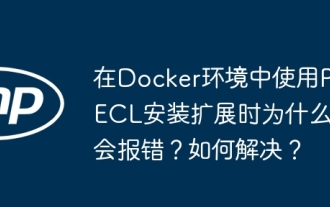
Causes and solutions for errors when using PECL to install extensions in Docker environment When using Docker environment, we often encounter some headaches...
